
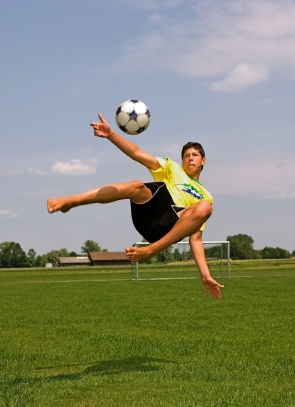
Skill or chance?
In the previous article A League Table Lottery we began exploring the treacherous territory of league tables by looking at the National Lottery. The underlying process was random in that case, yet a league table based on its outcomes still showed up potentially misleading patterns. This time we look at tables based on non-random processes, representing some of the most important and the most emotive league tables we come across: performance tables for schools and hospitals, or the football league, for example. Yet, as football fans will know, especially those backing losing teams, chance has a hand in these areas too, as success or failure can depend on a range of random factors. How informative are league tables in these cases, and can the inherent uncertainty be quantified? We'll investigate using the example of the Premier League.
The Premier League is the main English football league, with 20 teams each playing a home and away game against each of the others, making 38 matches for each team in a season, and 380 matches altogether. Teams are awarded 3 points for a win, 1 point for a draw and 0 points for losing. The league position is decided on total points. If two teams have an equal number of points, the ranking will be decided by goal difference: the number of goals scored minus the number of goals conceived. At the end of the season the top team carries off the trophy while the bottom three teams are relegated to a lower league.
We can use the history of the Premier League table over the 2006-2007 season to see how the spread of points develops.
The table below shows the points accumulated by the teams in the 2006-2007 season.
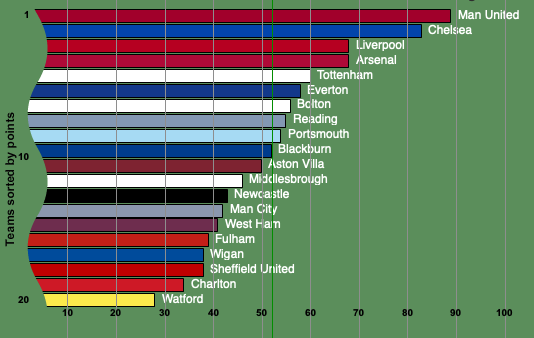
Manchester United won that particular season while Watford, Charlton Athletic and Sheffield United were relegated. To see to what extent the final table reflects the teams' quality, we'll compare it to a table produced by chance alone.
What distribution of points would you get by chance alone?
The spread of points at a particular time in the season can be visualised by a histogram. On the horizontal axis mark all the total scores a team could possibly have achieved so far. These range from 0 to the maximal number resulting from a team winning all its matches up to this point. Against each of these possible scores, plot the number of teams that have achieved that particular score.
Now assume that the league is decided purely by chance: all teams are of equal standard and the matches are decided at random. It's possible to work out a theoretical distribution which reflects the spread of points you'd expect assuming randomness (a little more on this follows below). The figure below again shows the 2006-2007 league table, but this time we have included the corresponding histogram (in grey) and the theoretical distribution (in white) at the bottm. It's clear that the actual and theoretical distributions differ quite strongly — we can conclude that there are genuine differences between the teams. To find out to what extent these difference are reflected in the table, we have to use some basic probability theory and statistics.
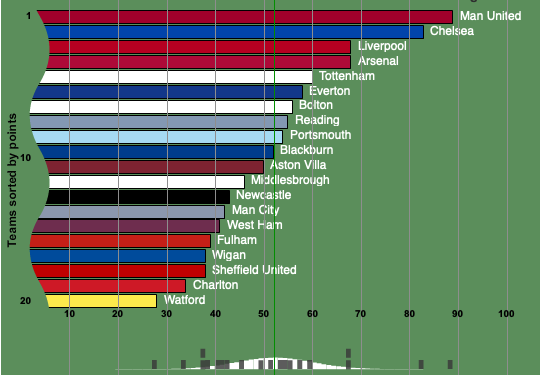
For each, the actual league and the chance league, we'll look at two quantities: the mean, describing the average number of points per team, and the variance, describing the spread of points around this average. The two variances are the numbers we will compare, as they will tell us to what extent the observed spread is a result of chance. \ \ For the actual results of
the 2006-2007 season the sample mean
To get some actual numbers for these values, we have a look at past records. These indicate that overall 48% of matches are home wins, 26% draws, and 26% are away wins — this will be called the 48/26/26 law. We can use these values to estimate the probabilities, so
How sure can we be about the true quality of each team?
The end-of-season point totals describe how well each team has performed, but since chance did have a considerable part to play, they do not quite reflect the true underlying quality of each team. There is a way, however, to gain more information from our league table.
For each individual team, the average number of points per match only gives a glimpse of the team's quality: we'd get a much better idea if the team had played, say, 38,000 or 380,000 matches, rather than just 38. Let's therefore imagine that the season continues indefinitely and that after an infinite number of matches we can work out the average, or mean number
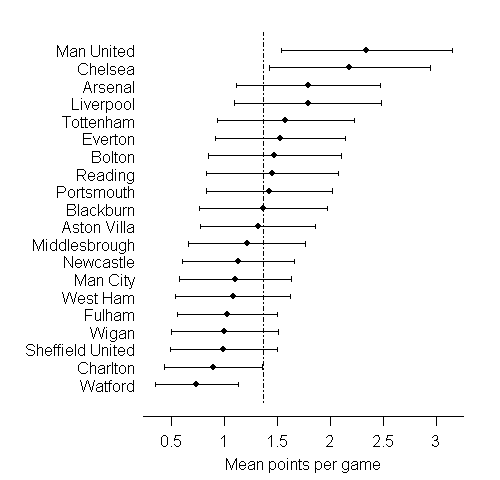
How sure can we be about the appropriate rank of each team?
Once we take the final average number of points per game as an estimate of an unknown quality measure, it becomes reasonable to view the observed rank of the team in the league table as an estimate of the true underlying rank of the team. Computer simulations from distributions based on the intervals shown above allow us to make predictions of thousands of "possible worlds" in each of which we can imagine the league fixtures going on and on until we are really certain of the "true ranking" of each team. The variability in these possible true rankings can be summarised as an interval around the current observed rank. The table below shows the teams on the vertical axis, plotted against their rank on the horizontal axis. For each team, we are 95% confident that the true rank lies in the interval shown.
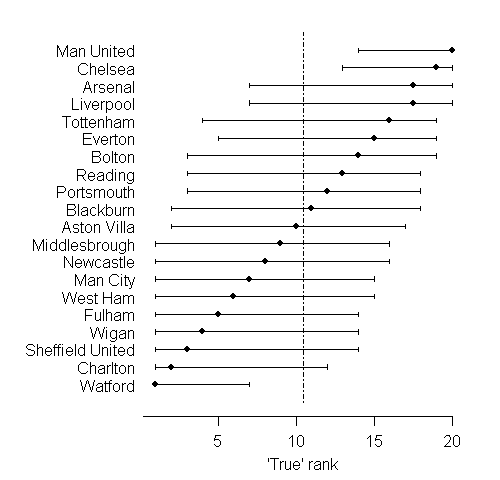
There is huge uncertainty as to the true ranks of the teams: this is typical of many applications of league tables. Manchester United and Chelsea again come up as the only teams we can be reasonably sure are in the top half of the table, while only Watford can be confidently placed in the bottom half.
We can also consider the probability that the season's winner, Manchester United, really was the best team: this works out to be 53%, compared to 31% for Chelsea. This could be interpreted as the probability that Chelsea would actually end up top of the league table were the season to continue indefinitely.
Were the teams that were relegated really the three worst teams? The probability of being one of the bottom three teams works out as 77% for Watford, 47% for Charlton Athletic, and 30% for Sheffield United. Wigan and Fulham narrowly escaped relegation, and in fact each has a 28% probability of truly being one of the bottom three teams.
The lesson is simple: if we are going to use league tables as a true measure of quality, then we have to serve them up with a lot more statistical information than is usually given.
About this article
Many more examples about the way that chance comes into sport can found in Beating the Odds: The Hidden Mathematics of Sport by Plus authors Rob Eastaway and John Haigh.
![]() |
David Spiegelhalter is Winton Professor of the Public Understanding of Risk at the University of Cambridge. Mike, David and the rest of their team have created the Understanding uncertainty website to inform the public about the mathematics of chance, risk, luck, uncertainty and probability. |
![]() |