Maths in a minute: Correlation versus causation
If your cat is wet when it comes in from outside then it's probably raining. Unless the cat likes to go swimming in the garden pond, that's a pretty solid conclusion to make.
What we have here is an example of correlation: the events of rain and cats getting wet are correlated, in other words, they tend to occur together. It is also an example of causation: the rain causes roaming cats to get wet. In this example the correlation is due to causation.
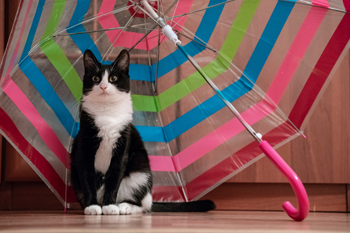
Do cats cause umbrellas?
However, this isn't always the case. When outdoor cats are getting wet you are also likely to see people walking around with umbrellas. Wet cats and umbrella carrying are correlated events, but neither do wet cats cause people to carry umbrellas, nor do umbrellas cause wet cats. In this case, correlation doesn't indicate causation. Instead, there's a third underlying phenomenon, rain, that causes them both.
It's pretty obvious in this example that correlation and causation aren't necessarily the same thing. There are many instances, however, where it's not that obvious. This can lead to misleading conclusions that can have serious effects.
For a particularly emotive example, think of immigration. Imagine two areas of the country, one which has a high proportion of immigrants and one which has a low one. If the area with a high immigrant proportion also records a higher crime rate, then you might jump to the conclusion that immigrants commit more crimes. In reality though, there might be other reasons for why the crime rates between areas differ. For example, a high density of people and higher levels of deprivation both tend to come with higher crime rates. To make any statement at all about immigration and crime, you need to dig a lot deeper. (We took this example from the website of The Migration Observatory at the University of Oxford.)
It's also important to remember that sometimes things are correlated by pure chance. There's a hilarious collection of examples on the spurious correlations website. It includes an apparent correlation between the per capita consumption of cheese in the US and the number of people who died by becoming entangled in their bedsheets.
.png)
Figure taken from the spurious correlations website. Data sources: U.S. Department of Agriculture and Centers for Disease Control & Prevention. CC BY 4.0.
The first thing a statistician would do when confronted with a correlation of any kind is to see if it is statistically significant — that is, to calculate how likely it is that the correlation you're seeing in the data you are looking at (cheese consumption and bedsheet deaths over the years in question) occurred by pure chance. Only when the correlation is statistically significant, so it looks like it isn't just a fluke, should you go on to investigate where it may come from — all the while keeping in mind that it may still be a fluke and that it may not indicate causation. You can find out more about correlation in the statistical sense on Towards data science.
In the meantime you can safely keep eating cheese without fear of dying by bedsheet.