
Understanding how our genes affect our lives is a complicated business, and takes cutting-edge science. However, by taking a typical mathematical approach, microbiologist and mathematician Johan Paulsson from Cambridge University has made things a little simpler.
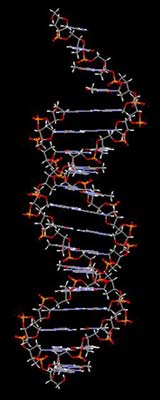
DNA, the blue-print for our whole body
A number of different molecules inside our cells are involved in acting on the information stored in our DNA. The information on a gene (a stretch of DNA) is copied, or transcribed, onto an mRNA molecule, which is then translated into a protein specified by the recipe on the mRNA. Particular proteins are required to catalyse each step in this process, and to facilitate the replication of DNA itself (read more about the process at Botany online). The interactions between all these molecules affect how the information encoded on our DNA is finally expressed as proteins. For example, scientists have discovered that the absence of certain other genes affects the ability of the insulin gene to trigger the production of insulin, resulting in diabetes in people missing those genes (read more from Harvard University).
All chemical reactions are probabilistic, partly due to randomness of the motion and ensuing collisions of the molecules in a reaction. This means that the amounts of the molecules involved (DNA, mRNA and proteins) in the reactions in a cell will vary randomly over time, producing noisy results when monitoring the levels of these molecules. And as the levels of one molecule can directly affect another - for example, making proteins requires mRNA - the noise in the levels of some molecules can be caused in part by "inheriting" the fluctuations of another.
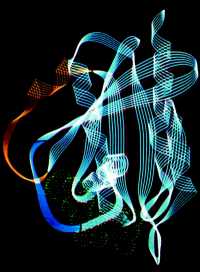
Picturing proteins. Image from U.S. Department of Energy Human Genome Program
Mathematical models such as Markov processes, where the current state of a system only depends on the immediate past, have been used to try to understand what is occurring inside our cells, including accounting for the noise seen experimentally (read about another application of Markov processes on Plus). So far each model has been tailor-made to the specific type of gene or protein examined. But Paulsson, like most microbiologists, is interested in the general principles that occur across all these cases. Therefore, like any good mathematician, he asked the question: "What is the simplest model that covers all these special cases?"
Rather than starting with a specific system in mind, Paulsson considered how the levels of one molecule (molecule A, say) would vary in a random environment created by just one other molecule (molecule B). For example, the creation of proteins is affected by the fluctuations in mRNA. In a recent paper in Nature, he modelled the system using a birth-and-death Markov process where the size of population B affects the rate at which population A increases or decreases (the number of mRNAs affect the production of proteins), but the size of population A does not affect the rate at which B increases.
Paulsson developed a generic equation that describes the noise in the levels of molecule A (e.g. proteins) in terms of its intrinsic noise (the random births and deaths of the protein) and the extrinsic noise from the environment provided by molecule B (e.g. the mRNA that is translated into the protein). The equation can be expressed in terms of natural parameters that are often easy to estimate, such as the average number and life span of the molecules, and the logarithmic gain - the effect of molecule B on the population change of molecule A.
Surprisingly, Paulsson discovered that this simple two-molecule model was strong enough to explain all the results that have been published so far, and that they could all be expressed as specific cases of his generic system. "It is definitely possible to draw concrete conclusions without first making very concrete assumptions, something that is considered almost oxymoronic in the field," he says. Although Paulsson doesn't rule out the possibility that things might have to get more complicated in the future, he hopes the work demonstrates "the practical usefulness of general theory in an area of research that has become dominated by tailor-made models".