Is causality fundamental?
Smoking causes cancer. Water causes the ground to be wet.
We may broadly agree on these statements, but what is this thing, "cause"? This issue has remained unsolved for thousands of years. Philosophers still argue over whether causality is truly a fundamental part of the world's structure, or whether intelligent beings create a sense of causality from a world of coincidences. The answer has implications that go beyond philosophy, however, as scientists and engineers attempt to improve how artificial intelligences (AI) reason and make decisions.
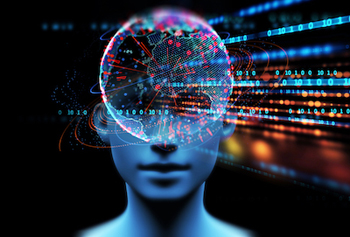
Foundational work on causality may reveal the kind of things AI can reason about and how they can make sense of reality. Image: monsitj
"Causation is at the heart of all human endeavour," says Sally Shrapnel, a medical doctor, physicist and biomedical scientist at the University of Queensland in Brisbane, Australia, who works at the interface of causality and machine learning. "It's about the effective means for bringing about change. What will happen if I do this rather than that?" says Shrapnel. "It's hard to function day-to-day without thinking causally."
A motivation of the early work on mathematical models of causality was the desire for an AI that would make decisions on complex medical treatments and drug dosages. Such an AI would have to deal with questions that are easy for a human to parse, but may be tougher for AI, such as: "If we had prescribed 10 mg of drug A instead of 20 mg of drug B, would the patient have recovered sooner?" The calculations needed to make the decision become vastly harder as the variables increase, but AI researchers have a framework in place that enables them to mathematically track how we as humans connect events into a cohesive story of cause-and-effect.
However, physicists are discovering that this well-used framework in AI research falls apart when faced with quantum mechanics — the set of laws that governs the behaviour of particles on microscopic scales. Now, two independent groups are attempting to uncover what's going wrong with the model, and whether it can be fixed. Their aim is to better understand how the causality we experience in day-to-day life may emerge from the fundamental quantum level. Along the way, they hope such foundational questions could help to improve the algorithms used to build better thinking machines in the future — and tell us just what kind of reasoning is possible for an AI.
Cause-and-effect connections
The current AI framework for understanding causality was pioneered by Israeli-American computer scientist Judea Pearl, in the 1990s. Pearl, now based at University of California, Los Angeles, wanted to explain how an intelligent creature would think and learn. His framework is basically a diagram of the cause-and-effect relations in the world, plus instructions for how a thinking system would uncover the diagram's connections. Those instructions explain how the thinking agent interacts with the world around it. According to the theory, interactions give the agent information about probabilities between events — what it, subjectively, thinks about the likelihood of various cause-and-effect connections.
Pearl's model is highly flexible. According to Shrapnel, it works and has uses in just about every branch of science — except quantum mechanics. "It works for all but one domain — and that happens to be our best understanding of the micro world," she says.
Now, most AI applications do not involve quantum processes, so this gap in Pearl's model isn't too much of a practical hindrance. However, if we want to get to grips with what causality truly is — and how it arises from a fundamental level — we need to find out why the framework breaks down on the microscale. That will help to illuminate human causal reasoning and, in turn, the potential for, and limitations of, AI reasoning.
The problem is that the story of how events are connected to each other in quantum mechanics is radically different than in the classical physics that governs our everyday lives. In pre-quantum thinking, a correlation between events usually means that events have causes in common. If we know everything about those common causes, then we know everything about the relations between the events. When it rains in your neighbourhood, then your lawn gets wet. If your lawn and your neighbour's lawn are both wet, then it probably rained last night. There is some kind of relationship between the rain and the wet lawns; we know that the one thing tends to happen along with the other things.
But in quantum mechanics, we have a new ingredient: quantum entanglement. Entanglement is a quantum property that allows events to have mysterious connections — correlations that can't fully be explained in terms of common causes with the classical rules of causality. Physicist Eric Cavalcanti of Griffith University, in Queensland, Australia, has been investigating how Pearl's classical causal framework fares when trying to capture this and other quantum processes.
Weird meteorology
Physicists routinely demonstrate entanglement in the lab. They create a pair of electrons, for instance, in an entangled state, in such a way that if they measure one particle's momentum and find it to be travelling at a certain speed in a particular direction, they immediately know that its entangled partner will have the same speed, but it will be moving in the opposite direction. They can't predict, before they measure it, whether the first electron will be moving due East, or due South. In fact, it's only at the point that they measure it, that the electron's direction is set. But they do know that whatever direction it snaps to, its partner electron will immediately snap into moving in the opposite direction. It's as if you know your neighbour's lawn will always be wet when yours is dry, and vice-versa — but you cannot predict which one will be wet and which will be dry, until you look and you can't ever explain how they got that way in terms of some common (but weird) meteorology.
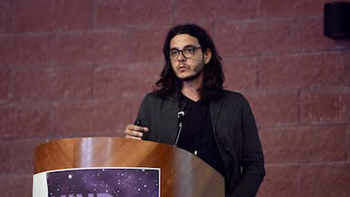
Eric Cavalcanti, Griffith University.
This new aspect to correlations messes up Pearl's algorithms, and Cavalcanti has tried to pinpoint exactly what goes wrong. In 2019, Cavalcanti and student J. C. Pearl proved that such peculiar quantum effects defy explanation via classical causality as set out by Judea Pearl's framework, unless you add extra correlations to the model that we could never detect (see this research paper). That would violate a principle of the whole set-up: that we can determine the actual causal structure that makes up our world.
Matthew Hoban of Goldsmiths, University of London, UK, was also inspired by some of the paradoxes about causality that entanglement appears to raise. "I had heard this stuff as an undergrad that we don't talk about," says Hoban. "I felt betrayed. We should talk about it, it's the most profound."
In a separate project, Hoban and Ana Belén Sainz at Gdansk University, Poland, are working out if Pearl's classical framework — and with it our everyday experience of the world — could develop from a primordial quantum causal scheme. Why, Sainz and Hoban ask, would agents in a quantum world come to think, as we do, that the world around them looks classical? They are examining the possible options at the micro level, that would predict a causal model like Pearl's at the macro level. "Given that daily life is classical," Sainz says, "what can we expect?"
Stranger than quantum
Sainz and Hoban have been using the causal framework to consider hypothetical rival models of fundamental physics that are even stranger than quantum theory, with correlations between particles that are even stronger than the level of entanglement seen in the lab (see this research paper). One puzzle for physicists is that these more bizarre alternatives to quantum theory could also be physically realised, in theory; but in practice, these models don't seem to be at work in our Universe. Why not? It's possible that only certain kinds of microscopic theories might be compatible with the right kind of macroscopic theory that leads to the world we see and experience.
For Sainz and Hoban, the crucial issue is the interplay between observer-dependent experience — subjectivity — and the external, objective world. The distinction between these two things is not always clear in a quantum world. Quantum physicists working in the lab still grapple with the question of where the system ends and the observer begins. Indeed, Cavalcanti has also been looking into ways to test whether or not observers are quantum objects that themselves become entangled in experiments (see this research paper).
Pearl's scheme tries to convey this interaction between the subjective and the objective through the instructions an agent follows when it interacts with the world around it. Both projects see one element of these instructions as a key part of the puzzle: counterfactuals. These are the sticky questions that ask what would have happened if events had only been different. If it hadn't rained last night, would your yard still be wet this morning? The example given above that can cause AI such headaches — "If we had prescribed 10 mg of drug A instead of 20 mg of drug B, would the patient have recovered sooner?" — is also a counterfactual.
Counterfactuals stand out because they don't obviously fit well into a quantum model. In quantum mechanics, so much about a particle is fundamentally unknowable until after we measure it. So a question like, "what would have happened if I had not measured the momentum of that electron," may not have an answer.
The key to modifying Pearl's method, says Cavalcanti, is to clarify "What are the kinds of counterfactuals that do make sense? What kind don't? What kind should not be asked? What kind should?"
Shrapnel agrees that understanding causality at a quantum level is important. She notes, though, that to be widely useful, the projects' results will likely need to go beyond Pearl's particular model of causality and embrace newer developments in causality research.
Cavalcanti suspects causality might not be a perfectly objective piece of the world that exists outside our own experiences. Instead, he thinks it emerges as a tool for rational agents to describe the complicated web of worldly activity that they witness. The web of correlations between groups of events — this thing seems to happen whenever that thing happens — coalesce into a causal story. Pearl's framework allows us to model this process for a wide range of phenomena, he says, so it is worth adapting to work with quantum mechanics. Such foundational work on causality may then reveal the kind of tasks to which AI are best suited. It could "help clarify what kinds of events AI can reason about?" he says. "How can AI reason to make sense of their reality?"
About this article
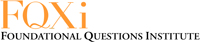
This article first appeared on the FQXi community website, which does for physics and cosmology what Plus does for maths: provide the public with a deeper understanding of known and future discoveries in these areas, and their potential implications for our worldview.