
Many aspects of our lives today are possible thanks to machine learning – where a machine is trained to do a specific, yet complex, job. We no longer think twice about speaking to our digital devices, clicking on recommended products from online stores, or using language translation apps and websites.
Since the 1980s neural networks have been used as the mathematical model for machine learning. Inspired by the structure of our brains, each "neuron" is a simple mathematical calculation, taking numbers as input and producing a single number as an output. Originally the neural networks consisted of just one or two layers of neurons due to the computational complexity of the training process. But since the early 2000s deep neural networks consisting of many layers have been possible, and are now used for tasks that vary from pre-screening job applications to revolutionary approaches in health care.
Deep learning is increasingly important in many areas both outside and inside science. Its usefulness has been proven, but there still are a lot of unanswered questions about the theory of why such deep learning approaches work. And that is why the Isaac Newton Institute (INI) in Cambridge is running a research programme called Mathematics of deep learning (MDL) which aims to understand the mathematical foundations of deep learning.
Empirical success
"The list of applications where deep learning is used successfully is so vast that it would probably be more efficient to list the areas where it's not used!" says Anders Hansen, from the University of Cambridge. Probably the most famous application of deep learning is in image classification, for example automatically determining if there's a dog or a cat in an image. But this is a far from trivial application, as any sort of automatic process which replaces the human vision and decision system is crucial in new technologies such as self-driving cars.
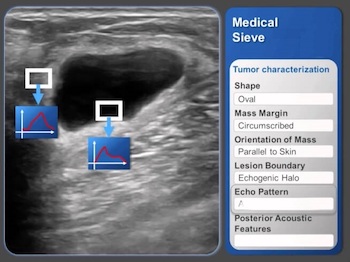
Interface of Medical Sieve, an algorithm by IBM for assisting in clinical decisions. (Image: The Medical Futurist – CC BY 4.0
Machine learning is now impacting healthcare, for example a computer analysing an MRI image and determining if you have cancer – assisting or potentially replacing a radiologist. "This is not science fiction," says Hansen. "Automatic diagnosis in medicine was already approved by the American Food and Drug Administration (FDA) in 2018."
There have been impressive results, says Carola Schönlieb, also from the University of Cambridge, but we don't understand why the results are so good and how they work. "This is a problem especially when you want to use deep learning methods to solve sensitive decision problems like in health care or for automated driving. Mathematics is really crucial to change this and is the key to opening the black box."
Building trust
The main theme of the programme is to develop a mathematical foundation for deep learning, says Gitta Kutyniok, from LMU Munich. "We all know how successful deep learning is in [its applications in] public life, sciences and mathematics. But most of the research is empirical and there's a great need for developing a theoretical foundation."
Regulatory organisations around the world are now having to consider how to regulate the growing use of machine learning and artificial intelligence (AI). The European Commission has already put forward suggestions on regulating the robustness and trustworthiness of AI systems in April 2021. A stronger mathematical foundation for deep learning would provide a language to assess these properties, impacting both the political and legal aspects of the use of this technology.
"Trustworthiness is a very important question in applications like medical diagnosis," says Peter Bartlett, from the University of California, Berkeley. A deep learning algorithm may be very successful, but it's vital you can quantify the uncertainties of when the algorithm's predictions are more or less accurate. "Assigning an appropriate statistical confidence to the prediction is hugely important and really lacking with many of these methods."
Deep mathematics
Mathematics may be key to assessing how confident we can be about deep learning, but the empirical successes of deep learning also pose some important mathematical questions. "Some of the really exciting, the big challenges, are understanding, from the mathematical perspective, how these methods are so successful," says Barlett. For example, relatively simple approaches in deep learning, such as first order gradient methods applied to very complex systems, are very successful at solving what should be very difficult optimisation problems. "Why are these simple approaches reliably leading to good solutions? Trying to understand why that is true from a theoretical perspective is really interesting. I think it's very exciting, these are genuinely new phenomena the practitioners have unearthed."
There are also exciting opportunities for deep learning to solve hard mathematical questions, with new applications constantly emerging in different fields. "First inverse problems in imaging science was a main focus, then around 2017 partial differential equations were thought to be solved by neural networks," says Kutyniok. One of the most recent areas deep learning is impacting in mathematics is in pure mathematics. "Machine learning is now used in computer assisted proof for determining counterexamples in, say, graph theory," says Hansen. "We see [deep learning applications] emerging in so many different areas, this is really what is super exciting."
This huge range of impacts, in applications in science and technology, and across many fields of mathematics, means that building this mathematical foundation will require input from many different areas. "One fascinating thing is that it basically requires all of mathematics, from approximation theory, to partial differential equations, even people from algebraic geometry are entering this field," says Kutyniok. "One needs to develop new mathematics to tackle the challenges. We hope this programme will bring together a very diverse group with different skills and expertise, to set the ground for developing new methods."
This article accompanies this video from the INI, where Dan Aspel spoke to the organisers – Anders Hansen (University of Cambridge), Gitta Kutyniok (LMU Munich), Peter Bartlett (University of California, Berkeley), and Carola Schönlieb (University of Cambridge) – of the Mathematics of Deep Learning programme, running at the INI from July-Dec 2021.
You can read more about the maths of deep learning here.
This article was produced as part of our collaboration with the Isaac Newton Institute for Mathematical Sciences (INI) – you can find all the content from the collaboration here.
The INI is an international research centre and our neighbour here on the University of Cambridge's maths campus. It attracts leading mathematical scientists from all over the world, and is open to all. Visit www.newton.ac.uk to find out more.
