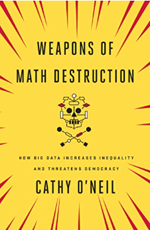
Weapons of math destruction: How big data increases inequality and threatens democracy
by Cathy O'Neil
In Weapons of math destruction Cathy O'Neil, mathematician-turned-Wall Street quant-turned-activist, offers a sobering glimpse into how algorithms dictate our lives at every turn. Following the life trajectory of a hypothetical citizen of the digital age, she offers a rich array of case studies that reveal the systematised injustices behind college admissions, incarceration, employment, insurance premiums, and campaigning. Each of these areas, she argues, are increasingly swayed by "weapons of math destruction", or WMDs: mathematical models that are opaque, unfair, and massively scalable. Often, the modeller begins with good intentions, but one way or another – through unconscious biases, a preference for efficiency over fairness, or simply poor statistical reasoning – the model perpetuates and exacerbates the existing inequalities and injustices in society.
O'Neil opens the book with a broad discussion of what constitutes a model, and what separates good ones from bad ones. Her skillful use of examples, which she demonstrates throughout the book, shines first here. By scrutinising the statistical models that baseball team managers use to recruit players, and the fluid conceptual models that parents use to decide which meals to put on the table, O'Neil shows how models are inherently subjective, require relevant (not just lots of) data, and can yield nonsense if applied too widely. These are not new ideas, but she presents them concisely, entertainingly, and with such clarity that they lay a solid foundation for her arguments in the rest of the book.
Crucially, O'Neil reinforces the notion that humans are, and should be, at the heart of the modelling process. Models are necessarily subjective, she claims, and this is ultimately a good thing. In fact, forgetting this subjectivity, twisting it, or intentionally hiding it, often leads to the most damaging models. She claims that notions of justice and compassion elude direct quantification, and so are usually ignored as models become more systematised. When this happens, the model's output must be scrutinised with special care; otherwise, these "softer" values risk being trampled in the name of efficiency. The autobiographical chapter (Shell shocked) demonstrates her deep concern for the people whose lives are most vulnerable to the misuse of big data. O'Neil's conversion from hedge fund analyst to activist hinged on her recognition of the fact that models should serve humanity, and never the reverse.
O'Neil's tone throughout the book is familiar, biting, and wry. In the beginning, her bravado risks making her claims sound exaggerated, but as evidence piles in chapter after chapter, the reader begins to share her exasperation. She boldly identifies the most egregious violators of modelling practice models by name. In doing so, she holds true to her own principles – if the reader is to develop a sound conceptual model of how algorithms dictate our lives, s/he needs specific data.
This book serves as an entry point into a nascent debate on the ethics of mathematics and the shortfalls of data analysis. It would be strengthened if it more explicitly pointed the reader to other resources on ethics in mathematics, and to the people and organisations dedicated to it. O'Neil, to her credit, doesn't even cite her own blog, which is a trove of resources on responsible data analysis. Her footnotes offer a start, but it would be helpful to have had a formal chapter pointing out the ethicists, analysts, and policy-makers who are advocating for the proper use of big data. She doesn't shy away from pointing out the villains in the fight – she should cite its heroes, too.
The book concludes on a note of hope. O'Neil notes that same algorithms that identify the poor and marginalised as potential money-making opportunities can also help to link people with the information and services they need most. After a near-relentless exposition of the harms caused by WMDs, the final few case studies paint a possible future in which mathematics, and mathematicians, work to serve people, not wealth. This is perhaps O'Neil's greatest achievement: in the wake of the dehumanisation she notes as a central outcome of the unfettered use of WMDs, she affirms that the human person, with her/his ability to adapt to new circumstances and weigh unquantifiable values like justice and compassion, can and must lie at the centre of mathematics. This book is an entertaining and eye-opening read for those who create algorithms and for those whose lives are in any way dictated by them – in short, for us all.
- Book details:
- Weapons of math destruction: How big data increases inequality and threatens democracy
- Cathy O’Neil
- paperback — 272 pages
- Allen Lane (2016)
- ISBN 978-0241296813
About the author
Stephen Kissler is a PhD candidate in applied mathematics at the University of Cambridge. He studies mathematical biology, currently focusing on how infectious diseases (primarily influenza) spread.
Weapons of Math Destruction
This is an important and highly readable book. To me, the most interesting idea is how all good intentions have unintended consequences. Why not take for example the book itself. It has been written by a professional mathematician, published by a world-famous publishing house and two well-known math professors have said few words of praise. In spite of that, the only formula in the book – the one explaining the much criticized Body Mass Index (page 176 of the Penguin edition) is wrong. What an illustration of the concept of unintended consequences.
Models in theoretical macroeconomics
I have been examining this subject for some time and have devised a way of simplifying what first seems to be a most complex situation into one that is simple but not too simple (Einstein). Those interested may like to see: SSRN 2865571 "Einstein's Criterion Applied to Logical Macroeconomics Modeling".