Could AI could provide judges with objective information to help them make fairer, and more transparent, decisions about sentencing? As we saw in the first part of this article there are issues of bias in using AI to learn from existing case data. If algorithms can't learn to produce fair outputs from existing case data, then perhaps they need to be told how to be fair by the people who build them — to be designed in such a way that outputs adhere to our notion of fairness. To do this we'd first need to understand this notion ourselves.
Building in fairness
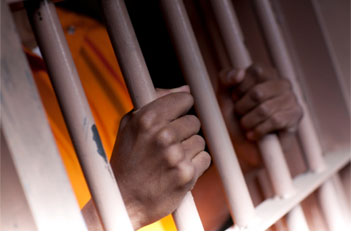
What's proportional for one judge may not be proportional for another.
In law, fairness is to a large extent built around the concepts of consistency (that every person be treated equally) and proportionality (that the sentence be proportional to the seriousness of the crime). There aren't, however, clear definitions of these concepts. "The concepts are quite nebulous, the way they are interpreted through the courts can change over time," says Tiarks. This isn't necessarily a bad thing, she points out, as it allows interpretation to change along with social norms.
The nebulousness is compounded by the fact that it's up to individual judges to decide what the main purpose of a sentence should be: to punish the offender, or to rehabilitate them. (There are in fact five statutory purposes of sentencing, whose relative importance is up to the judge.) In a 2021 paper Tiarks gives the example of an offender who had seriously injured someone with a hammer. The original sentence imposed by the Crown Court was a three year community order with requirements for mental health treatment and supervision. The Court of Appeal later substituted this by a five year prison sentence. The respective judges felt differently about what the sentence should achieve — and this affected their idea of what was proportional.
When it comes to consistency, individual cases can vary widely in their particulars, so it's hard to come up with hard and fast rules for how certain factors should be taken into account. Think of intoxication, for example. It's usually held as an aggravating factor, but can also be seen as diminishing an offender's responsibility.
Given these ambiguities, the Maths for Justice study group found no ready way of translating a notion of fairness into something that would work in an algorithm, so more work would be needed to achieve this. It recommended approaching the task from multiple angles to try and capture the multi-faceted nature of the concepts involved.
Mathematics also facilitates deeper insights into notions of proportionality and consistency one can perhaps draw inspiration from. "This could lead us into some interesting directions in the future, to see how owing to mathematics and data science, combined with legal and societal reflection, we could have improved measures of consistency,” says study group participant Michał Kubiak from the Centre for Industrial Applications of Mathematics and Systems Engineering (Poland). Kubiak also has a background in industry and policy as a fellow at the European DIGITAL SME Alliance.
There is also another important factor of fairness we haven't yet mentioned: transparency, which allows people to understand why a sentence was given. The study group did not directly investigate the impact of algorithms on transparency, but some questions readily spring to mind. One is whether people would be able to understand how an algorithm arrives at its outputs. Not only because AI algorithms are complex and have a tendency to function as black boxes, but also because there may not be sufficient information in the public domain. The (non-AI) COMPAS algorithm used in the US to assess risk of reoffending, for example, is kept secret by its developer. In addition, argues Tiarks, it would not be clear to what extent an algorithm might sway a judge.
Overall, if AI sentencing methods are eventually used, the Maths for Justice study group advised a flexible approach, with algorithms being frequently reviewed, updated, and redeveloped, and there always being a possibility of appeal to humans. Some of this, the group suggested, may require a new framework of legal bodies.
Setting the scene and low-hanging fruit
Assuming that calls for the use of AI in sentencing will only get louder, Tiarks suggests that now is a good time to create the landscape that offers the best possible starting conditions. Apart from setting mathematicians and data scientists to work on the problems involved, this is also about making sure we systematically collect relevant data from the cases that are passing through the courts, and that such data is consistently available to judges.
Ironically, the existing use of algorithms, such as those used in OASys, proves a bit of a stumbling block here. Originally the algorithm was meant to form part of wide-ranging and holistic pre-sentence reports about offenders, written by probation officers. "With the courts being more pressed for time and money, the vast majority of pre-sentence reports are now short-form reports," says Tiarks. "Often the risk score [provided by the algorithm] appears independently without much context about the offender. This is a problem if we're looking at incorporating machine learning into the process, because we are getting less useful data." Sorting out the data situation, Tiarks says, "is going to take an injection of money into the criminal justice system."
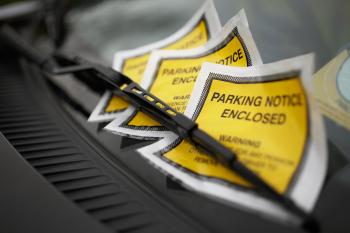
In the meantime, Tiarks and Kubiak point to less spectacular, but less controversial, ways in which AI could help. UK law is based on precedent, which means that courts are bound by previous decisions. As a result, judges and lawyers are forever searching huge databases of historic cases. This is something which the large language models that power applications such as ChatGPT are well-suited to do. "There is already AI-enabled case search, it's just not available to everyone," says Tiarks. "In legal research AI could be really useful and relatively uncontroversial."
Other low hanging fruit might involve crimes where sentencing is nearly automatic already and there's a deluge of data, such as parking offences. "Back office type of work is something that AI could improve," says Kubiak. "However it is important to note that these applications currently also come with their own technical and moral quandaries, such as hallucinations (AI models making up wildly erroneous outputs under certain conditions) or issues with keeping the private data confidential."
Zooming out to the general picture, Kubiak points out that the criminal justice system doesn't operate in a bubble. AI is permeating our lives from many different directions and people's use and understanding of it is changing. Recently, controversy ensued over whether or not availability of Wikipedia articles on certain previous rulings could influence sentencing in Ireland. With AI we might uncover more unexpected interactions of this type.
This means there is a lot to learn from other fields where ethics and practicalities are also an issue, concerning the use of AI in filtering job or benefit applications, for example, or risks from autonomous weapon systems. It also means that a lot more work can be done. "One of the first things to investigate, I think, is how AI will change wider technical and social norms and how it'll change the environment in which the criminal justice system operates," says Kubiak.
Both Tiarks and Kubiak believe there's nothing inevitable about AI in sentencing — it's up to people to decide if and how to use it. But if we do, Kubiak says, then "it's really important to have good ethical, scientific and systemic research, and consult all stakeholders at every step of the way."
There's also another point to remember, says Kubiak: even if we do achieve completely unbiased sentencing algorithms, this does not automatically guarantee fair outcomes for everyone who passes through the criminal justice system. Human and systemic bias from other sources is likely to remain, adding yet another layer of complexity to an already complex issue.
About this article
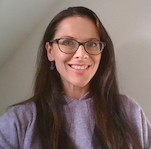
Elizabeth Tiarks is a Lecturer in Criminal Law and Criminal Justice at Aberdeen University. Her research currently focuses on the use of AI in criminal justice, particularly sentencing. She previously practised as a criminal barrister in Newcastle Upon Tyne and remains an academic member of New Park Court Chambers.
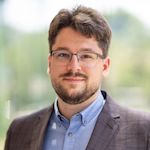
Michał Kubiak is a policy fellow at the European DIGITAL SME Alliance and an alumnus of the Centre for Industrial Applications of Mathematics and Systems Engineering (Poland). Outside of STEM-related work he is a professional choir singer.
Marianne Freiberger, Editor of Plus, interviewed Tiarks and Kubiak in December 2023 following the Maths for Justice virtual study group organised by the Virtual Forum for Knowledge Exchange in Mathematical Sciences (V-KEMS). She is very grateful for Tiarks' and Kubiak's help with this article.
This article was produced as part of our collaborations with the Isaac Newton Institute for Mathematical Sciences (INI), the Newton Gateway to Mathematics and the Mathematics for Deep Learning (Maths4DL) research programme.
The INI is an international research centre and our neighbour here on the University of Cambridge's maths campus. The Newton Gateway is the impact initiative of the INI, which engages with users of mathematics. You can find all the content from the collaboration here.
Maths4DL brings together researchers from the universities of Bath and Cambridge, and University College London and aims to combine theory, modelling, data and computation to unlock the next generation of deep learning. You can see more content produced with Maths4DL here.


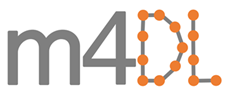