
Brief summary
There have been suggestions that AI may be used in sentencing to ensure sentences are fair. A recent study group involving law experts and mathematicians has pin-pointed a number of challenges that arise from this idea.
Artificial intelligence is making inroads into all areas of life, and the criminal justice system is likely to be no exception. One area that has been taken into consideration for AI is sentencing. Like all humans, judges can be biassed and their decisions appear inconsistent. Algorithms could, perhaps, be more objective and transparent. They could also ease the workload for humans, a benefit for a system strapped for cash and time.
Sentencing is not something we would want to meddle with lightly, however. "Sentencing is one of the most intrusive powers of the state," says Elizabeth Tiarks, a Lecturer in Criminal Law and Criminal Justice at Aberdeen University. "It has the potential to impact on the offender and the victim, their families and dependants, and also the public."
Seeing if, and how, artificial intelligence could help would benefit from a multi-disciplinary approach. Ideally it would involve not only experts in law and ethics, but also mathematicians, statisticians and data scientists, who understand the algorithms and the data. This is why the Virtual Forum for Knowledge Exchange in Mathematical Sciences (V-KEMS) recently ran the Maths for Justice virtual study group which brought together experts from these different fields, including Tiarks. Together the participants began to unpick a number of issues surrounding the use of AI in sentencing.
Bias in the system
In England and Wales the Lammy review, published in 2017, brought disparities in the criminal justice system to the forefront of political debate and raised suspicions of bias. Although people from Black, Asian, and Ethnic Minority (BAME) backgrounds make up only 14% of the overall population, the review pointed out they make up 25% of prisoners. In relation to drug offences, it highlighted that the odds of receiving a prison sentence are around 240% higher for BAME offenders than for White offenders.
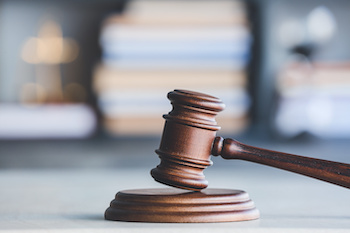
Various further studies support the idea that some of the disparities are down to unfair treatment. A 2020 research report from the Sentencing Council and a 2022 study from Administrative Data Research UK, for example, suggest that ethnic disparities persist, even when more stringent statistical efforts are made to only compare sentences for crimes of similar severity. The Sentencing Council study also found a gender bias, with men tending to receive harsher sentences than women.
Findings like these are behind the calls for the use of algorithms in sentencing. Algorithms could help, not necessarily by replacing judges altogether, but by providing outputs that can feed into judges' considerations.
Indeed, algorithms have already been involved in sentencing for some time. In England and Wales the Offender Assessment System (OASys) contains an algorithmic component which scores the risk of an offender reoffending. The score goes into pre-sentence reports submitted to judges. The algorithm has been used since the late 1990s, attracting little controversy or scrutiny. But this algorithm can't be deemed intelligent. It's an old-fashioned list of instructions for calculating a score. A human could also follow these.
Baking in bias
When we talk about artificial intelligence we mean machine learning or, more specifically, deep learning algorithms. These are different from the old-fashioned sort, not only because they're a lot more powerful.
As their name suggests, rather than being given an explicit list of instructions, machine learning algorithms are able to learn. They are trained on existing data that provide examples of the kind of outputs the algorithm is expected to deliver given a particular input (find out more in this brief introduction). In a naive application of machine learning algorithms to sentencing, this training data would consist of information about past offenders, their offences, and the sentences they received.
During the training phase, the algorithm would spot patterns within this data, which it would then use to suggest sentences (or perhaps deliver risk scores) for offenders it hasn't seen before. As a simplified example, if the existing data showed that armed robbery always comes with a ten year prison sentence, then the algorithm would pick this up and suggest a ten year prison term whenever confronted with a bank robber in the future. In practice, though, the patterns spotted by an algorithm can be complex and even hidden from human view. In this case, it may be impossible for a human to understand why the algorithm came up with a particular output — the algorithm would function as a black box.
One concern about applying this approach to sentencing is that the data sets used for training may be less than perfect, missing important, or containing erroneous, information. Another is that, if training data contains bias, then the algorithm trained on it will learn this bias. It may pick up, for example, that BAME people get harsher sentences than White people, or that men get harsher sentences than women. Bias would be baked into the algorithm. "If our starting point is that judicial decision-making is problematic, then why would we train our algorithms on problematic decisions," says Tiarks.
Bias could be learned even if information about offenders' ethnic background, or gender, is removed from the training data as far as possible. There may be other pieces of information within the training data that are highly correlated to a person's ethnic background or gender — information about personal circumstances, or socio-economic or geographical data, for example. If the algorithm links this information to harsher sentences, then it has in effect also linked the ethnic background, or gender, to harsher sentences.
Confounding factors
To get around this problem you'd have to strip bias from training data in cleverer ways or make sure the algorithm is optimally equipped to deal with it in other ways. But this would involve knowing exactly where the bias comes from and what it entails, and that can be tricky. A famous example here is the hungry judge effect. A 2011 study of parole board decisions in Israel found that judges were considerably more likely to give lenient sentences at the beginning of a session than just before the break, suggesting that hungry judges are harsher (or that "justice is what the judge had for breakfast").
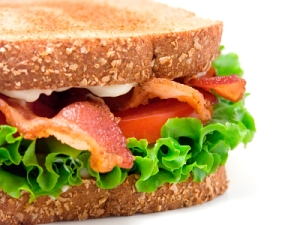
Do hungry judges give harsher sentences?
But the effect can also be explained in other ways. For example, when an offender ends up with a tough sentence, then this may mean the case was relatively straight-forward: a guilty plea, no doubt, no mitigating circumstances. Such cases are likely to be scheduled at the end of a session to avoid over-running. The comparative leniency at the start of a session may therefore be down to the ordering of cases, rather than the stomach of the judge.
The hungry judge effect is an instance of correlation being confused with causation, which can happen in lots of other ways too. Coming back to UK data, it's known that offenders from BAME backgrounds are less likely to enter a guilty plea than White offenders. A guilty plea can significantly reduce a sentence, so perhaps judges are, to an extent at least, responding to the lack of a guilty plea when sentencing offenders from BAME backgrounds (indeed the 2022 study from ADRUK suggests this). A reluctance to enter a guilty plea may of course itself be down to systemic racism — the Lammy review assigns it to a lack of faith in police officers or solicitors — but the harsher sentence cannot automatically and in all cases be blamed on a judge being racist themselves.
An offender's socio-economic status could also have a confounding effect. Class and ethnicity are interrelated, not least because ethnic minorities are generally more deprived than the White majority. Some of the bias in sentencing may be down to judges being biassed against class rather than race. Alternatively, it may be down to other factors, such as poor people being unable to afford expensive lawyers. There are US studies (such as a 2021 study by Ellen Donnelly) which support the idea that class and ethnicity are interlinked when it comes to bias in sentencing. More work is needed, and indeed, another strand of the Maths for Justice study group investigated ways of approaching the problem. Participant Jose Pina-Sánchez and his colleagues have recently suggested a far-ranging analysis to explore the issue further.
Overall, there isn't a clear consensus on how biassed judges really are and what they are biassed against. "There is a lot of uncertainty around how big the problem actually is," says Tiarks. "The question is whether we can make a reasonable assessment of it."
Such an assessment was also recommended by David Lammy, author of the Lammy review, so that action can be taken if the bias is found to be intolerable, or the population reassured if it's not (as Lammy put it, "explain or reform"). It is even more important, argues Tiarks, if the bias of judges is the main justification for using data-driven AI in sentencing.
Building in fairness
If algorithms can't learn to produce fair outputs from existing case data, then perhaps they need to be told how to be fair by the people who build them — to be designed in such a way that outputs adhere to our notion of fairness. To do this we'd first need to understand this notion ourselves. To find out how we might approach this issue, see the next page.
About this article
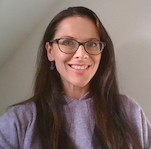
Elizabeth Tiarks is a Lecturer in Criminal Law and Criminal Justice at Aberdeen University. Her research currently focuses on the use of AI in criminal justice, particularly sentencing. She previously practised as a criminal barrister in Newcastle Upon Tyne and remains an academic member of New Park Court Chambers.
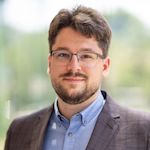
Michał Kubiak is a policy fellow at the European DIGITAL SME Alliance and an alumnus of the Centre for Industrial Applications of Mathematics and Systems Engineering (Poland). Outside of STEM-related work he is a professional choir singer.
Marianne Freiberger, Editor of Plus, interviewed Tiarks and Kubiak in December 2023 following the Maths for Justice virtual study group organised by the Virtual Forum for Knowledge Exchange in Mathematical Sciences (V-KEMS). She is very grateful for Tiarks' and Kubiak's help with this article.
This article was produced as part of our collaborations with the Isaac Newton Institute for Mathematical Sciences (INI), the Newton Gateway to Mathematics and the Mathematics for Deep Learning (Maths4DL) research programme.
The INI is an international research centre and our neighbour here on the University of Cambridge's maths campus. The Newton Gateway is the impact initiative of the INI, which engages with users of mathematics. You can find all the content from the collaboration here.
Maths4DL brings together researchers from the universities of Bath and Cambridge, and University College London and aims to combine theory, modelling, data and computation to unlock the next generation of deep learning. You can see more content produced with Maths4DL here.


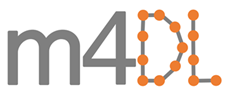