The idea that there are fundamental laws that describe the physical universe is now a part of everyday culture. We expect that scientists can predict how a physical system will behave using these mathematical descriptions, whether it be the trajectory of a football, the chain reaction in a nuclear power station or how to bounce signals between mobile phones.
"Ya canna break the laws of physics, Captain!"
(Scotty, Star Trek)
What many people might not realise is that the same cannot be said for biology. "Biology is not yet a predictive science, there are essentially no fundamental laws," says Thomas Fink, a physicist working on the DARPA Fundamental Laws of Biology program (FunBio). "But the exciting thing is that biology appears to be on the cusp of bifurcating into an experimental branch and a theoretical branch. Are there underlying fundamental laws? Is there an equivalent of [Newton's second law of motion], F=ma, in biology? While we can't yet say for sure, there is increasing evidence that there are deep unifying mathematical principles."
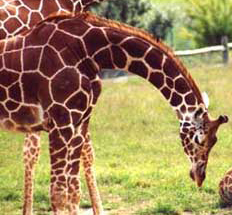
Beautiful mathematics
Mathematics has played a part in the development of the biological sciences and has even led mathematicians to push the limits of their own subjects, such as Alan Turing's pioneering work developing reaction-diffusion equations to explain animal patterns. However, Fink says that biology, in terms of maturity, is at the stage that physics was 300 years ago — we understand the pieces, the genes and the cells, but we still can't describe how they fit together into complex systems such as organs, organisms and ecosystems, much less how they evolve or how the brain works.
Fink and his colleagues on the FunBio program are trying to give biology a theoretical push. FunBio brings together people from many different fields — physics, mathematics and biology — to try to draw out the fundamental rules underpinning how biological systems work. "Is there a mathematically elegant story behind life? That's the question FunBio is trying to answer."
Are you ready to evolve?
"People who wish to analyse nature without using mathematics must settle for a reduced understanding."
(Richard Feynman, The Character of Physical Law, 1965)
One of the exciting areas they are working on is trying to understand the intricacies of evolution. We often think of life as evolving from some sort of primordial soup where the complex molecules necessary for life first came together. But Fink believes that more than half of the story happened before that moment — just which primordial soups have the right stuff to be able to evolve, and how did they come about?
"Everyone says the standard model for evolution is mutation, selection and inheritance. Put those ingredients together in a box and you get evolution. But the reality is, when we put those things into models of evolution, or set up appropriate systems of artificial life, we just don't get life-like evolution — we don't find the evolution of complex, surprising things. Some fundamental is missing. What gives a system the capacity to evolve? What makes a system evolvable?"

An RNA sequence folds into a specific shape determined by which parts of the sequence align.
Why are some biological systems more suited to evolution than others? Fink and his colleagues are exploring this question, and their starting point is a model of RNA folding. RNA molecules read the genetic information contained in DNA in order to produce proteins in a cell (you can read more about RNA on Plus). You can think of RNA sequences as strings consisting of the four letters U, G, C and A, representing the different nucleotides (the molecules that make up RNA and DNA). These genetic strings don't float about like a long piece of seaweed in the ocean: each RNA sequence folds itself into a specific shape. The letters in different stretches of the sequence line up next to each other (technically speaking their base pairs are complementary) and loops and bends are formed between the aligned segments.
If you look at a short segment of RNA, say 30 letters long, you have 430 possible different sequences. Each sequence folds into exactly one shape but many other sequences might also fold into the same shape. So you have fewer shapes (about 1.830 possible shapes for RNA sequences of length 30) than sequences.
RNA folding is an insightful framework for studying evolvability. The different sequences are called genotypes and the different shapes they fold into are called phenotypes. "The phenotype is the end-product that somehow the environment notices," says Fink. "The genotype is the particular way you made it. The environment doesn't notice the sequence; it notices the particular fold you've got. "
To mathematically model a biological system of genotypes and phenotypes, for example a population of individual organisms and their generations of offspring, we first draw a point for each particular RNA sequence (or genotype) that occurs in the system. We can make a graph on these points by connecting any two sequences that differ by just one point mutation, just one spelling error. For example, the sequences ...AACUG... and ...AACUA... are almost the same, they differ by only one letter, and so we draw an edge between them.

A simple mutation graph. The vertices represent the different RNA sequences or genotypes that are linked by an edge if they differ by one letter. The vertices are coloured according to their pheonotype, the shape the sequence folds into.
The result is called a mutation graph and represents the possible ways the biological system can evolve: making one point mutation at a time traces a path through this graph. The genotypes are the points, or vertices, in the graph. Now let's paint each point (each sequence) a colour, corresponding to which shape the sequence folds into. These colours are the phenotypes. All the sequences that fold into the same shape are represented by points of the same colour on the graph.
"So now we have this interesting mathematical object," says Fink. "We can make point mutations, which means we move along an edge of the graph. Some of these point mutations change our colour, change our phenotype: others do not. If we mutate but we don't change colour, we call that a neutral mutation. Now we can start to talk about different concepts: we can think about what it means to be robust, and what it means to be evolvable."
Connected clusters of points of the same colour represent these neutral mutations. For example the isolated purple vertex in the bottom left of the graph will change colour no matter how it mutates. However, the purple vertex at the right of the graph has a probability of 1/3 that it will stay purple.
You can define the robustness of a phenotype, say purple, by averaging the probability of staying purple, over all the purple points — how likely it is that you will remain the same shape from mutation to mutation. (For this simple graph the robustness of the purple phenotype is 4/15.) If you imagine the organism lives in an environment where any phenotype other than purple is lethal, the robustness of the system is how likely new generations are to be the purple phenotype and therefore survive.
But suppose now the environment changes and it may now be more beneficial to be a different phenotype. In this case you are interested in your evolvability — how many different phenotypes you can access through mutation. In terms of the graph, the isolated purple vertex at the bottom left of the graph only has access to one other phenotype, red. However, the cluster of four connected purple points across the middle of the graph have access to both the red and green phenotypes.
"A biological system is robust if a change in genotype does not lead to a change in phenotype. The system is evolvable if a particular phenotype has access to many other phenotypes. Until recently, these two qualities have appeared to be, paradoxically, opposed: if changes in genotypes don't lead to changes in phenotypes, the system is robust; if they do lead to changes in phenotypes, the system is evolvable." Recent work, notably that of the theoretical biologist Günter Wagner, suggests that actual biological systems are delicately tuned so that robustness and evolvability are in fact correlated — the more evolvable the system the more robust it is — this has been explicitly studied in the RNA-folding model described above. "Systems which are both robust and evolvable are capable of extensive exploration of the phenotypic landscape in light of changes to the environment, and can thus try out new mechanisms without destroying core functionality."
"This is a simple model but there are a number of mysteries here," says Fink. "For instance, when does increasing your evolvability correspond to increasing your robustness? Are there critical phenomena here when the system suddenly, in a jump transition, becomes very evolvable and you can access almost all other types of phenotypes?"
From his current mathematical analysis of mutation graphs, Fink suspects that for a biological system to be both robust and evolvable, it might not have to be as delicately tuned as is presently believed. "It appears that the number of phenotypes needs to be sufficiently small compared to the number of genotypes, and this may change the system in some critical way. There may be a jump transition in the evolvability of the system, strongly related to critical phenomena in percolation theory [see below]. Is that a special property of living systems? Or is it actually something deeper than that? "
Can biology lead to new theorems?
"The lack of real contact between mathematics and biology is either a tragedy, a scandal, or a challenge, it is hard to decide which." (Gian-Carlo Rota, Discrete Thoughts, 1986)
It is clear that mathematical know-how is needed to transform biology into a predictive, theoretical science. The problem is that contributing to biological discovery alone is not enough to draw in the best physicists and mathematicians. "I'm not interested in biology per se," says Fink. "If I was, I'd be a biologist. I'm interested in using beautiful mathematics to describe the world around me. If understanding life on earth needs beautiful mathematics then I want to get involved."
So if biologists only turn to mathematicians and physicists to help them solve mundane mathematical problems or do data analysis, these theorists won't be excited. Fink jokes that when a biologist asks him to simply analyse their data, he asks them to mow his lawn — to him the two tasks are equally fascinating. "It's got to be a two-way process — biological insight achieved through elegant mathematics. The culture of biological research is only beginning to appreciate this — that an elegant theory is more likely to be a true theory, even in the life sciences."
One example of this two-way interaction was when Fink, Francis Brown and PhD student Karen Willbrand brought their mathematical perspective to a problem in medical genomics and ended up proving a new result in number theory. They were looking at the data produced by a microarray study of bladder tumours done by experimentalists at the Curie Institute. "The basic idea is that a pathologist puts 20 different tumour samples in order of how advanced they are," explains Fink. "For each tumour sample you've got 30,000 genes, and a microarray measures the concentration of each one." This gives you 30,000 curves, one curve for each gene; and each curve has 20 points, one point for each of the tumours. Most of the curves look random, but very occasionally one appears to have a recognisable pattern: "Hey, this curve starts off high, then slowly moves down, then shoots up again. Wow! Indicator gene! We've got a predictor for bladder cancer," says Fink, imagining the reaction of a biologist conducting the study.
"The problem is, to be able to know what is interesting, one needs to know what is boring," explains Fink. "Is it a likely thing or an unlikely thing to find a curve that starts of going down, down, down, then finishes going up, up, up? What if we find one among 30,000 curves, is that a surprise?" A theoretical understanding of how a random curve typically behaves will not only tell us whether a curve that looks interesting really is interesting, but also helps pinpoint curves that don't look interesting but in fact are.
Instead of trying to understand the biology, Fink and his collaborators focused on the maths. Imagine you have a curve of 5 data points, say, 0.77, 0.84, 0.51, 0.30, 0.26. If you connect the points with line segments you'll see that the first segment is increasing, and the next three segments are decreasing. So the curve goes up, down, down, down, and the curve's so-called up-down signature is + - - -. The question is, is this curve unusual and therefore something to shout about if it shows up in an experiment?

Probability P(σ) of finding a random curve with up–down signature σ, for 2, 3 and 4 data points. You can think of curves of, say, three points, as permutations of the set 1, 2 and 3. The permutation 3 2 1 has the up-down signature or - -, while the permutations 3 1 2 and 2 1 3 both have the same up-down signature of - +.
To answer this question, Fink and his collaborators calculated the probability of a randomly generated permutation (say a random arrangement of the numbers 1, 2, 3, 4 and 5) having the same up-down signature. (The theory of up-down signatures is the same for random curves and random permutations.) If a permutation is very unlikely to have that shape by chance alone, then the curve, and hence the gene, is likely to be biologically significant. However, the distribution of up-down signatures over random permutations is an unsolved problem in mathematics. "It turns out to be a nice combinatorics problem that people started looking at about 130 years ago," says Fink. The problem was first studied by D. André in 1881, when he calculated the probability that a permutation has an alternating up-down signature (+ - + - + - …). Fink and collaborators generalised this result for arbitrary signatures, which led to their number theory results.
Back in the original biological context, their technique provides a way of blindly identifying biologically important genes from microarray experiments, without any prior assumptions about what sort of behaviour to expect. As a benchmark, they tested their technique on well-studied yeast cell cycle experiments (you can read more in their paper). It has since been used in current research including studies on other forms of cancer.
On the brink of new mathematics
Fink believes the mathematical discoveries from theoretical biology are only just beginning and there is a lot more yet to be found. "The view among many physicists is that biology today is like quantum mechanics in the twentieth century. There is huge virgin territory, and people are racing in to make discoveries. There's a lot of low lying fruit, whereas in more mature fields like particle physics you've got to climb up high."
Tell us what you think!
Do you think that biology can become a theoretical predictive science? You can also leave a comment at the end of this article to voice your thoughts.
Charting this new mathematical territory of theoretical biology is starting to excite the mathematical community. Bernd Sturmfels, also part of the FunBio programme, has asked in his 2008 Clay lecture: "Will a theoretical biologist ever win a Fields medal [one of the highest hounours in mathematics]?" Finks says "Many theoretical physicists have won Fields medals and the boundary between physics and mathematics is almost imperceptible. Is the lack of real contact between mathematics and biology a thing of the past?"
One encouraging sign is that the work of the 2010 Field's medallist, Stanislav Smirnov , is already linked, indirectly, to the mutation graphs and evolvability described earlier. Understanding the connected clusters of phenotypes on mutation graphs can be posed as a problem in percolation theory — the area of statistical physics in which Smirnov works . "In a randomly coloured mutation graph, if the number of phenotypes is small enough, the typical size of a connected phenotype cluster will be sufficiently large to have access to lots of other phenotypes," says Fink. "So if you are in a shifting environment you can move into that phenotype if you need to. And percolation theory asks: do you have lots of isolated little components or is there one giant component that spans the whole system?"
So perhaps the mathematical world will start to recognise the rich pickings in theoretical biology, and it's only a matter of time before we report on a theoretical biologist winning a Fields medal. The fundamental laws of biology may not be as far off as they seem.
Further reading:
You can read more about Fink's work on theoretical biology on his website. You can also find out about the fundamental laws of physics in Symmetry rules and the maths behind the biomedical sciences on Plus.
About this article

Thomas Fink
Thomas Fink is a theoretical physicist at the Curie Institute and the London Institute for Mathematical Sciences. He uses statistical mechanics to study complex systems in physics and interdisciplinary fields. His research interests include discrete dynamics, complex networks and fundamental laws of biology. Thomas has also written two popular books: The Man's Book, an almanac for men; and The 85 Ways to Tie a Tie, a book about ties and tie knots.
Rachel Thomas is co-editor of Plus. She interviewed Thomas Fink in London in September 2010.
Comments
Biology's next microscope
Rather surprising that there was no reference to the article that this piece was clearly influenced by:
http://www.plosbiology.org/article/info:doi/10.1371/journal.pbio.0020439
Gib
What an enjoyable article!
I found this very interesting and Fink's feelings about Biologists made me smile as I have encountered much the same attitude when trying to work with biologists (as someone from a mathematical background).