
The type of artificial intelligence that has been making so much progress in recent years is based on something called machine learning. This approach relies purely on data — for example, by looking through the information contained in lots of images of cats and dogs, a machine learning algorithm can learn which patterns in the information are related to cats and which are related to dogs. It then uses this knowledge to decide whether a picture it has never seen before shows a cat or a dog.

Maths in a minute: Machine learning and neural networks — Find out more about how machine learning works in this brief article. You might also want to look at our artificial intelligence FAQ.
Machine learning works miraculously well. It's behind things like ChatGPT and all the amazing AI generated imagery and video you might have seen. But there are also applications beyond text and images. For example, the vast amounts of weather data that are continually being collected provide a fertile ground for machine learning algorithms. The algorithms could spot patterns within the data that humans may not be able to see, and potentially come up with more accurate weather forecasts.

Can artificial intelligence help predict the weather? — See this article to find out what AI can and cannot do when it comes to weather forecasting.
While this is a promising approach, AI is still some way away from replacing traditional methods in weather forecasting and also in other applications that involve the physical world. So why not give it a helping hand by enabling it to not just learn from data alone, but also take into account some of the vast knowledge of physics we humans have accumulated over millennia?
This is the idea behind physics-informed neural networks (PINNs for short). We learnt about PINNs from members of the Maths4DL research project with whom we are lucky enough to collaborate.

AI goes to physics class — Find out more about PINNs and why researchers are excited about them in this article, based on our conversations with Maths4DL members Chris Budd and Georg Maierhofer, and past member Rob Tovey. (And yes, there is a justification for using yet another image of a kitten).

How physics can help AI learn about the real world — You can also listen to our interview with Georg Maierhofer in this edition of our Maths on the move podcast.
PINNs are still in their infancy, but when they become good enough to implement, they could help with applications beyond weather forecasting, for example in engineering, biology and chemistry.
This content was produced as part of our collaboration with the Mathematics for Deep Learning (Maths4DL) research programme. Maths4DL brings together researchers from the universities of Bath and Cambridge, and University College London and aims to combine theory, modelling, data and computation to unlock the next generation of deep learning. You can see more content produced with Maths4DL here.
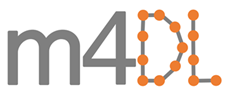