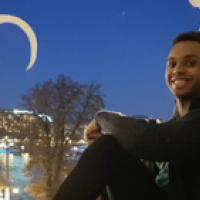
Teo Deveney is an early career researcher at the University of Bath and a member of the Maths4DL (mathematics for deep learning) research project. Here he tells us about his work in artificial intelligence, which comes with some great travel opportunities and inspiring moments in the pub.
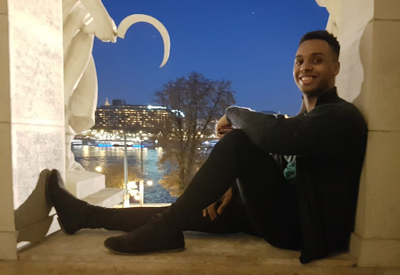
Teo Deveney
I work on topics at the interface of mathematics, statistics, and a form of artificial intelligence called deep learning. During my PhD I investigated how deep learning methods can be used to solve problems where both statistics and physics play a role.
As an example, suppose you want to know the direction the wind is blowing in. One thing you could do is blow some soap bubbles. If they all move away in one direction, then you guess that that's also the direction of the wind. To arrive at this answer you have used your knowledge of physics, namely that wind carries soap bubbles along with it. But you have also done a simple statistical analysis: you have collected a bunch of data points (the direction of travel of every soap bubble) and noted that all these data points are very similar.
This is the type of problem I looked at, though the physics isn't always so intuitive and can require a lot of computing power to work out. I used a statistical approach called Bayesian inference where the answer you get isn't precise but comes with some uncertainty. In my PhD I investigated how deep learning can be used to estimate this uncertainty.
I tend to think of my PhD as research in "deep learning for mathematics", in the sense that I looked at how deep learning can be used to solve mathematical problems. However since then I've been engaged with the converse, as a postdoc in "mathematics for deep learning". Here I'm motivated by how ideas from a range of mathematics can be applied to better perform deep learning tasks — for example I'm currently involved in a project where the same mathematics that you'd use to describe the movement of those soap bubbles is being applied to do things like generating AI artwork!
[You can find out more about deep learning in this article and about Bayesian statistics in this article.]
My interest in this area stems from a mixture of its mathematical breadth and the many applications it has in the real world. Different types of mathematics can feel quite different to one another, and traditionally researchers tend to focus heavily around certain topics. I find quite a wide range of mathematical topics interesting, and so I wanted to work in an area that encompassed and combined a variety of topics to produce theory and methods that have impact outside of mathematics.
For example, in my PhD I wanted to work with differential equations and physics, but also do the Bayesian statistics I mentioned above. Traditionally, Bayesian statisticians don't think much about physics and vice versa, though there seems to be a trend towards this type of thing changing. The area of mathematics for deep learning is an exciting and fast moving field that really is focused on progressing deep learning by leveraging and developing a range of relevant mathematics, so it was a perfect fit for my interests.
I really value collaboration, be that between mathematicians, academics in other fields, industrialists, or anyone else. I think us numerical scientists can do a lot to help others overcome challenges, but we often underestimate how the real world complexities of these challenges can help develop deeper mathematical insights that improve our own research. The Maths4DL group has a great collaborative atmosphere, led by some world experts at the intersections of mathematics and deeplearning, and in collaboration with industrial experts who are passionate about how research in the area can have real world impact.
We span three universities and so far I've spent significant stints in Bath and Cambridge. These cross-institution collaboration opportunities and exposure to different communities and working environments would be much more difficult in a more traditional postdoc position.
On a typical working day the first thing I do is take note of any meetings or teaching I might have lined up and set up a plan for the rest of the day. From here things can vary quite a lot depending on what the day demands. My research time is typically occupied by coding up ideas, doing handwritten theoretical work, writing up results, or preparing talks. Communication and collaboration with others in the community or the wider public is an important part of our role, so sometimes I'll travel to present my work, or work with industry or government. Aside from that, the mathematical communities I've been part of have always been friendly and I can often be found chatting over coffee or lunch, or getting involved in the social activities in the department.
My favourite mathematical moments often come at inconvenient times. An idea that became one of the most significant contributions to a paper was conceived in the pub. This idea allowed me to scale the technique I'd been working on to much larger problems and achieve a higher accuracy without needing a more powerful computer. It was really valuable to my work, though my friend would attest to how annoying it was when I proceeded to bore them by talking about it for the next hour. This has happened a few times over various projects, there are invariably stumbling blocks in research and the solutions don't always come at the most convenient times, but it makes them more memorable than the ones that are solved at a desk.
I've also had some really amazing moments that have happened as a result of mathematics, though I wouldn't call them mathematical moments. For example last year I spent some time in Santiago, Chile, working with the university and an astronomy company to improve their algorithms. Prior to this I've spent periods in Ulaanbaatar, Mongolia, and Asuncion, Paraguay, working with government and building statistical capacity. The participants get a lot from these events and I get to explore an interesting place I likely wouldn't otherwise visit. These experiences are incredibly rewarding!