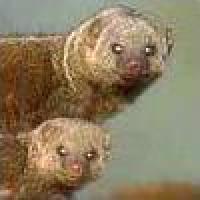
The essence of mathematical modelling is simplification. Natural events are the result of multiple interrelated processes, themselves dependent on a history of other processes, in an almost endless web of cause and effect. To study a system, the mathematical modeller begins by identifying the crucial aspects of the system, those that seem to characterize it. Initially, she need not concern herself with how such characteristics come to be, only with how to describe them in simple mathematical terms.
This is a very human approach. Many of us will have encountered it when learning a language, and probably even day to day while learning the universal language of mathematics. We may have been given examples of similar triangles or of imaginary numbers to play around with and get a feel for, before being taught the underlying theory. And you will no doubt be aware that as the mathematical journey continues you will uncover yet more subtle and profound detail behind the ideas as they were first presented.
The story of Dr. Moss and the mongooses
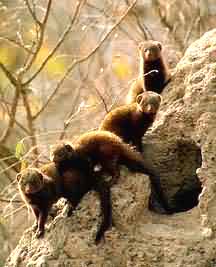
Going about their daily, mongoosey activities.
Picture copyright Spook Skelton
So it is with our mathematical modeller, Dr. Moss. At first, she will throw out many details of the system and concentrate on its most obvious characteristics. Let us take the example of modelling the spread of disease through a population of mongooses. Mongooses are an excellent example for our purposes because most people won't have a clue what they look like, where they live, or how they go
about their daily, mongoosey activities. All of these are vital pieces of information, you might think, and will surely influence any ideas Dr. Moss may form on the spread of disease through their population. Although you would be right to think like this, at such an early stage Dr. Moss is quite happy to ignore these details and consider instead the basic concepts of "a disease" which spreads
"somehow" between various "identical" members of "a population". As with that quiet glass of water on the table, the teeming life of the problem will only come into view if we later apply some magnification.
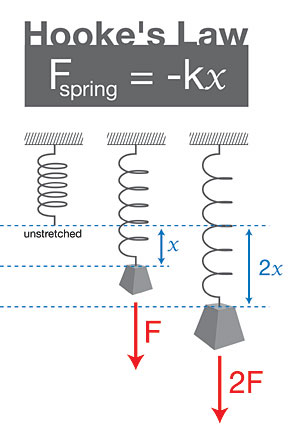
The concept of understanding a process by omitting the details is not a new one. Indeed, this could almost serve as a definition of science, and certainly this is how science has progressed since the Renaissance, and one could argue that sections of early Greek and Arabic sciences pioneered the approach. A crystal clear - if somewhat gory - example is provided by Robert Hooke, a British scientist of the late 17th century. You may have encountered Hooke's Law in physics: the tension in a spring is directly proportional to its extension. Like many learned men of his time (and as we sadly know, nearly all famous individuals of this time were men), Hooke had his thumb in many scientific and technological pies, being heavily involved in the already-influential Royal Society in London. Apart from investigating springs, air pumps, watches, telescopes, microscopes and much more besides, he also performed some truly awful experiments on animals. On one occasion he established the function of the lungs beyond reasonable doubt by opening up the chest of a dog and keeping it alive by pumping air into those organs. This reductionist approach to understanding, which aims at breaking complex phenomena down into component parts and seeking to understand these before integrating them into a coherent picture, is continued to this day. Despite having suffocated many animals in his air pump chambers (to investigate the effects of low air pressure on a living being) and vivisected others, even Hooke was reluctant to repeat the dog experiment, only doing so when others performed it so badly he could no longer stand the suffering of the creatures.
Dr. Moss, however, need not get her hands dirty. She begins by writing down very simple equations that she thinks contain the principal characteristics of the diseased mongoose population. These equations will involve both a rate of change of the proportion of the population succumbing to disease, and some unknown parameters, which we will consider shortly. The resulting equations will be differential equations. These are equations involving the rate of change of quantities either in time, or in space, or in both, and are a part of calculus.
Buffaloes per orange...
The equations Dr. Moss initially writes down will be independent of the particulars of the disease, geography, climate, and the mongooses themselves, and may serve other situations equally well. These specifics enter the parameters of the system, or influence it from its boundaries in time and space. It is here that levels of complexity and correspondence with the observed world begin to emerge.Firstly, note that we talk in terms of the proportion of the population currently tucked up with the mongoose equivalent of chicken soup. The idea is that it doesn't matter how many mongooses there are (beyond reasonable constraints: the spread of disease through a population of two mongooses is very dull), only the percentage of them that are sick, falling sick, recovering, and contagious. This is an example of normalising, and is an extremely useful trick.
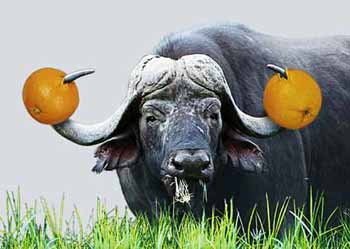
Another important point here is that the equations make sense in broad terms when they are reinterpreted. If she is not careful, Dr. Moss may end up writing a lovely equation that measures the spread of disease not in percentage per day, but in buffaloes per orange. Indeed, when she takes all such units out of the equation, she will be in one of two equally attractive positions. She may find that all the units disappear from the equations (they cancel exactly with each other) in which case she or anyone else is able to measure quantities in whichever units they favour - including buffaloes per orange. Alternatively, the units may gang up with each other in what are known as dimensionless groups. This means that the units are still explicitly present in the equations, but they can be gathered together into expressions which reduce to a single number with no units, so she is still free to measure in buffaloes per orange. Additionally, Dr Moss is able to consider different types of solution depending on the size of the dimensionless groups. This type of dimensional analysis is very powerful indeed and is also a great way to become famous: most dimensional groups are named after the person who popularised them.
Let us look over Dr. Moss's shoulder and consider an example of a dimensionless group in her mongoose problem. A gathering of units could well yield the dimensionless group SDCAM0/F, which we shall call Moss's number, where S is the mean susceptibility of each mongoose to the disease, D is the density of mongooses in their environment, C is the contagiousness of the disease, A is the mean area covered by a mongoose in his or her daily wanderings, M0 is the mean weight of a mongoose and F the amount of food available to each mongoose. Now, D is measured in "mongooses per unit area" and so has dimensions Length-2; S is a scalar (an ordinary dimensionless number); and so on. If we write down the units for each of these terms, all the dimensions cancel and what remains is therefore a dimensionless number.
It is clear that Moss's number is very important and immediately gives us plenty of qualitative information on the system. If the equation is such that the rate of spread of the disease is proportional to Moss's number, then when it is large we can see that the disease will rapidly spread through the population. If it is small, however, most mongooses will be safe. Looking at the number this makes sense: a large value could be telling us that the disease is highly infectious or that there is so little food that the mongooses are weak. A small number could indicate that there are so few mongooses that they rarely come into close contact and thus the disease spreads only slowly. There is a formal mathematical method known as asymptotic analysis that can be used to extract more quantitative results from considering large and small limits of dimensionless groups.
Back to reality
As she gets more of a feel for the system, and begins to see some results from the simplified model, Dr. Moss will build more and more realism into her model. She may try to include factors such as the age variation in the population, or how an individual's daily activities contribute to its likelihood of becoming infected. These factors will usually add further terms to the governing differential equation, or system of differential equations. Or they may change the initial or boundary conditions of the system - the data which filter in through the edges of the environment, or when the disease first appears.
Depending on the type of equations involved, such changes can alter the outcome dramatically. Linear equations are reasonably predictable: once you've found a solution for given initial and boundary conditions, you know the rest. An example we met earlier is Hooke's Law: if you double the length of the spring, you double its tension: simple. Nonlinear equations are entirely different beasts. They exhibit what is technically called chaos, though the name is a little misleading because their behaviour is not random - it is controlled by the equations, after all - but can be incredibly rich in detail. A great example of a nonlinear system exhibiting chaos is all around you: the weather. As with all chaotic systems, a tiny change in the initial conditions can have a dramatic effect: drop the temperature of a small patch of ocean by less than a degree and you could create a monsoon on the other side of the world. Imagine if extending a spring by just a small amount caused the tension to increase by a factor of 10, and a further slight extension produced an almost zero tension!
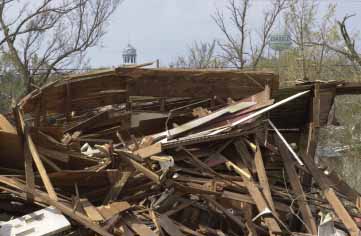
Chaos in every sense of the word: tornado damage
Photo copyright FEMA
It is probably fair to say that everything is controlled by nonlinear systems of equations, all borrowing data from one another and nudging each other into new solution regimes. But Dr. Moss will have looked first only at the linearised versions, gradually increasing the complexity and nonlinearity until greater and greater correspondence with reality was found. Some systems are so complicated that only linearised versions can be contemplated by hand, and Dr. Moss may decide ultimately to program her computer to try to solve the nonlinear equations.
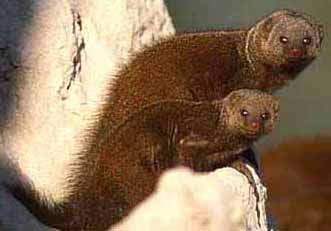
Mongooses - but will they come to the phone?
Image courtesy of African Wildlife Foundation, photo by Darryl and Sharna Balfour
Thus a mathematician has, in the comfort of her office, sat down and systematically worked through an ever-improving system of equations. She has started with the simplest possible case that captures features of the problem. Then, as she began to understand the dynamics of the problem, she will have talked to mongoose experts (that is, scientists whose expertise is mongooses: experts who are mongooses tend not to come to the telephone) and built more and more of the real world into her equations. Finally, she may have been able to characterize the phenomenology of the disease, and explain why perhaps small changes in apparently unrelated factors can yield wildly different prognoses for our mongooses.
The chances are she won't have needed to leave her office, and next week is another week.
About the author
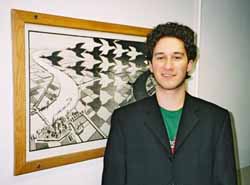
Phil Wilson gained the MSci in Mathematics from University College London in 2000. His interest in all areas of maths blossomed there, and he took courses in most disciplines, culminating in a thesis on the proof of Fermat's Last Theorem. He is currently nearing the end of his PhD at UCL, where he is studying the high-speed flow of air through convoluted ducts. He hopes to pursue a career in research and teaching.
He will be teaching Fluid Dynamics at UCL in the Autumn before starting a postdoctoral position modelling biological cell dynamics at Tokyo University.
This article now forms part of our coverage of the cutting-edge research done at the Isaac Newton Institute for Mathematical Sciences (INI) in Cambridge. The INI is an international research centre and our neighbour here on the University of Cambridge's maths campus. It attracts leading mathematical scientists from all over the world, and is open to all. Visit www.newton.ac.uk to find out more.
