
Back to the Do you know what's good for you package
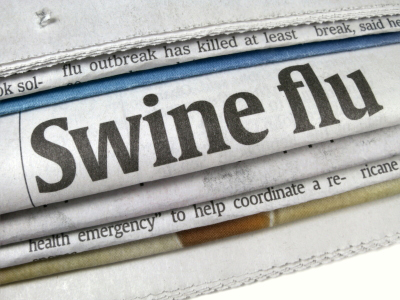
Swine flu: Real danger or media hype?
Just over two weeks after the outbreak of swine flu, sorry, H1N1, most of us have come round to the idea that a pandemic doesn't always necessitate panic. The infection is spreading steadily, but in most people it's relatively mild and only a very small number of people have died outside Mexico. In an editorial last week the medical journal The Lancet praised the British media, and the general public, for their "balanced and rational" reaction to the outbreak. Perhaps we're learning how to be on alert without losing our heads.
The Lancet was responding to a piece by the Guardian's Simon Jenkins (who has been previously noted on Plus) who dismissed initial coverage of the outbreak as exaggerated by media and scientists to sell papers and drugs. But according to The Lancet, Jenkins got hype confused with scientific uncertainty: in the face of the many unknowns that come with this new virus, scientists play out a range of different scenarios, including worst-case ones. If these don't happen, then this doesn't necessarily invalidate their methods — it might even mean that interventions made as a result of the predictions have worked.
But how do scientists make these predictions in the face of so many uncertainties? The biological side — understanding the virus and producing vaccines — is down to life scientists, but understanding how it will spread is a matter for mathematicians. They build mathematical models that mimic the progress of an infection. There is a range of tried-and-tested epidemiological models, but the devil is in the detail: the predictions of any one model depend crucially on the assumptions made about the nature of the virus. Hence the uncertainty.
Two times two times two...
As an example, imagine that a person infected with a new virus infects, on average, two other people a day. Starting with one sick person, we have two new sick people the next day, four new sick people the day after that, and so on. Assuming that people don't recover and become immune, it takes just over three weeks until the number of sick people is larger than the population of Britain. If a sick person infects three people a day on average, then the whole of Britain will be infected within just over two weeks. The exponential growth means that one extra infection a day per sick person makes the disease spread a lot faster.
One number does (almost) everything
The basic bit of information here — the average number of people a sick person will infect in a population that has no immunity against the virus — is the most important parameter in disease modelling. It's called the basic reproduction number of an infectious disease. It measures how fast the disease will spread, and, consequently, how hard it is to control, and it's therefore one of the first bits of information scientists will try and estimate when a new disease has broken out.
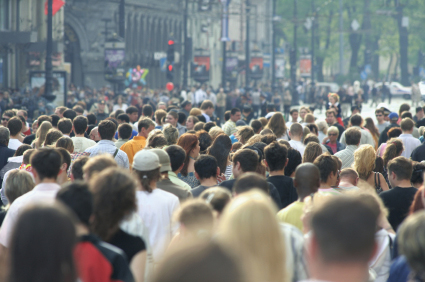
The reproductive number measures how fast a disease spreads.
Getting that estimate right is hugely important, but it's no easy feat, because data from the beginning of the outbreak isn't always readily available. The first analysis of H1N1 data from Mexico, published this week in Science, uses sophisticated mathematical machinery to make sense of incomplete information. The authors stress that "there are uncertainties about all aspects of this outbreak", and their estimate of the reproductive number for H1N1 is correspondingly vague, between 1.2 and 1.6. This compares to a value between 1.5 and 3 for normal seasonal influenza and between 12 and 18 for measles. The H1N1 number may seem low, but it's in the same range as reproduction numbers of previous flu pandemics. Assuming that the infection rate stays constant, only a reproduction number of less than 1 guarantees that the disease will die out of its own accord.
The reproduction number can also tell you quite a few things about possible interventions to control a disease. For example, it gives you a way of working out what proportion of the population should be vaccinated to prevent the infection from spiralling out of control. If an infected individual normally passes the disease to four others, then you should immunise just over 3/4 of the population: out of the four that would normally have been infected by one sick person, more than three would now be immune (on average), bringing the infection rate down to less than 1, and causing the disease to eventually die out. Similarly, for a general reproductive number R0, you can achieve so-called herd immunity by vaccinating a proportion of 1-1/R0 of the population.
Challenging models
Taking aside the difficulty of getting good estimates of important numbers, there's the question of how to model the spread of a disease. The simple exponential model above is far too crude, as it ignores the fact that people can recover, become immune, or die. Accordingly, the model predicts that any disease with a reproduction number greater than 1 will continue to spread forever.
More sophisticated models divide the population into groups. In the SIR model, for example, people are either susceptible to the disease (S), infected and contagious (I), or have recovered and are immune (R). Every day (or whatever the unit of time you're using) a certain number of susceptible people become infected and a certain number of infected people recover. For example, if b is the average number of contacts a person has a day and S is the proportion of the population that is susceptible to the disease, then an infected person meets bS susceptible people a day on average. Assuming that the disease is passed on to all of them, bSI people pass from the susceptible to the infected class every day, where I is the current number of infected people.
The number of people who pass from the infected to the recovered class is worked out in terms of the average number of days a person is sick. If this is equal to d, then the chance of recovery on a given day is 1/d, so out of I infected people I/d pass over into the recovered class every day.
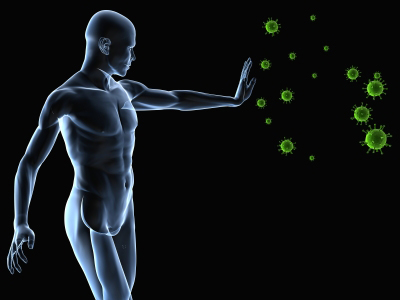
Mathematical models can be used to test interventions.
This simple model can be made more mathematical by rewriting it in terms of differential equations. Throwing in some general assumptions on the rate of births and deaths in the population, you can then run your model on a computer to simulate the long-term behaviour of the disease. All you need in this simple case are estimates of the average number of contacts a person has a day, b, and the average duration of the disease d. The reproductive number, according to this model, is bd/N, where N is the total size of the population. If this number is less than 1, then the disease will eventually die out. If it's greater than 1, then the model predicts that epidemics occur periodically for a while, until the proportion of infected people settles down to a constant value — the disease has become endemic.
The great thing about this type of compartmental model is that you can add extra compartments to make it more realistic. For example, you can add a group of people who are incubating the disease, but aren't yet contagious, or people who are contagious but don't yet show symptoms. You can also play around with the transition rates between compartments to predict the effect of interventions. For example, you can model the effect of antiviral drugs by reducing the average duration of the sickness, or you can predict the effect of quarantine by varying the contact rate.
Once you can mimic how a disease spreads between people who physically meet, you can combine this with models that describe people's travel behaviour to predict how and if a disease will spread around the globe. You can also use these more sophisticated models to find out what kind of interventions would be most effective. A study published last year, for example, used a compartmental model coupled with a travel model to show that traffic restrictions would have little effect on the evolution of a pandemic, a possible reason for the World Health Organisation's refusal to recommend a complete ban on travel in the face of H1N1. More encouragingly, the study found that treating 5% of the population with antiviral drugs would mitigate a pandemic for diseases with a reproductive number up to 1.9.
Effective as these epidemiological models may be, they are only ever as good as the underlying assumptions. If you're not sure about the basic characteristics of a virus, for example its reproductive number, then the predictions of any model will be necessarily vague. Even a relatively small change in one of the parameters can lead to significantly different predictions. Any good epidemiologist is explicit about the underlying assumptions of a model and the uncertainties concerning parameter estimates. But once the predictions hit the headlines, these caveats are often lost, leading casual readers to mistake uncertainty for falsity.
Further reading
- You can find out more about the SIR model in the Plus article The mathematics of diseases;
- The Motivate video conferencing project has a write-up of a project involving disease modelling. It contains a spreadsheet which allows you to change disease parameters and see how the model's predictions change.
Back to the Mathematics of infectious disease package