
Brief summary
Experts in public health, industry and disease modelling came together this summer to discuss how maths can help decision makers and industry prepare for the next pandemic.
Several speakers explored ways that mathematical modelling (mathematical descriptions of real world systems that allow us to study how these systems progress) can support the development and use of new vaccines against the next pandemic disease.
Since emerging from the pandemic, many of us would rather think about anything else. But experts in public health, industry and disease modelling are continuing to think about pandemics, both learning lessons from the last one, and preparing for the next one. This summer the JUNIPER partnership and the Newton Gateway to Mathematics brought many of them together at an event, called Preventing Future Pandemics, to discuss how maths can help decision makers and industry prepare for the next pandemic.
This event was part of a longer research programme at the Isaac Newton Institute (INI) that followed on from a special online research programme the INI held in 2020 in response to the COVID-19 pandemic. During the pandemic mathematical models, in particular epidemic models that mathematically describe how diseases progress during an outbreak, were vital in providing scientific advice. The event this summer brought together disease modellers with decision makers and industry experts to explore the key challenges to using modelling in helping prepare for the next pandemic.
The 100 Days Mission
"What if we had life-saving vaccines in 100 days?" asked Arminder Deol, from CEPI (Coalition for Epidemic Preparedness Innovations), one of the speakers at the event. Previous to the COVID-19 pandemic the fastest any vaccine had been developed and approved was four years. "COVID-19 vaccines however were advanced at record speeds, due to prior research on other coronaviruses and other innovations. But what if we could go even quicker?"
This is the 100 Days Mission of CEPI. CEPI began in 2017 as a global coalition of industry, governments and academics to accelerate the development of vaccines. As well as prioritising existing known diseases, CEPI also focuses on preparing for Disease X, a term introduced in 2018 by the World Health Organisation to describe a new or unidentified pathogen that could cause a serious epidemic or even the next pandemic. COVID-19 was the first example of Disease X.
If the COVID-19 vaccine had been developed in 100 days, by April 2020, then at that point we would have had only 2.3 million confirmed cases worldwide, as opposed to the almost 70 million cases that we had by 8 December (when the first vaccine became available). Mathematical modelling by a team at Imperial College London estimated that this would have saved 8.3 million people from dying from COVID-19, 26.7 million people going to hospital and 1.4 billion people from catching the disease. "The faster a vaccine can be developed and deployed, the faster a pandemic can be controlled and contained," said Deol. "The goal for CEPI is for the world to be able to respond to the next Disease X with a new vaccine in just 100 days."
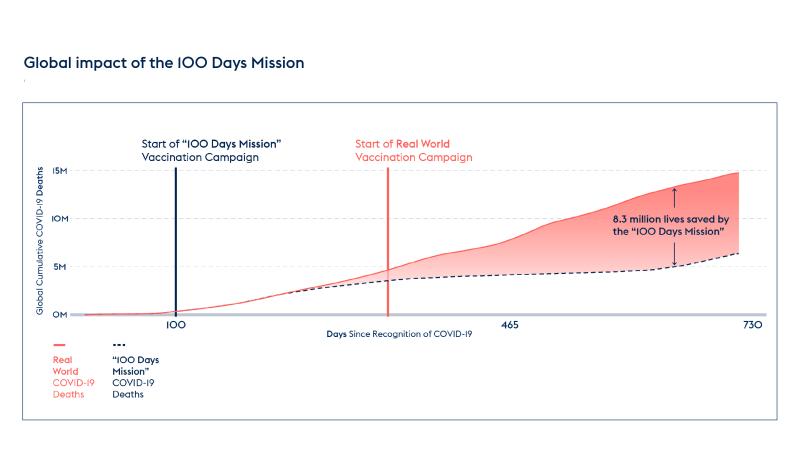
"CEPI likes to call the 100 Days Mission a moonshot mission – although it's ambitious it is technologically within reach," said Deol. CEPI has identified a number of areas of innovation that will improve the process of developing, testing and manufacturing vaccines, and improving surveillance worldwide. "Modelling can support the work in all these areas."
CEPI have identified families of viruses with the greatest potential to cause the next pandemic and are developing research and vaccine libraries for these diseases. For example there are currently no vaccines available for Lassa fever, a disease that causes thousands of deaths each year throughout West Africa. As well as supporting the development and testing of several different potential vaccines, CEPI also funds the Enable research programme to understand the current impact of the disease. And CEPI funded a recent study, led by JUNIPER members David Smith and Emily Nixon from the Universities of Oxford and Liverpool, that used mathematical modelling to estimate the personal, economic and societal costs that could be saved by having a vaccine for Lassa fever, and particularly a vaccine that could be available within 100 days of a new Lassa-X variant of the disease emerging. Such information is vital when deciding whether to fund a vaccination campaign within limited public health budgets.
Designing vaccines
"We are used to thinking of modelling once you have a vaccine, but modelling is useful throughout the vaccine development process," said Hannah Clapham, from the National University of Singapore, in her talk at the Preventing Future Pandemics event.
During development mathematical models can be used to optimise the design of the vaccine. Vaccines typically contain the maximum amount of antigen (the ingredient that stimulates production of antibodies to the virus) that is safe – you want the amount of antigen that maximises the effectiveness of the vaccine while minimising the risk of side effects. But mathematical modelling has shown that this isn't always optimal.

For example, in a paper from 2016, Sophie J. Rhodes and colleagues from the London School of Hygiene and Tropical Medicine produced models based on experimental data that describe the immune response of an individual to different doses of antigen in a tuberculosis vaccine. These dose response curves showed that the optimal dose, the one producing the highest immune response, was not always the maximum safe dose. The research suggests that such modelling should be used to identify the best dose when designing vaccines.
Testing vaccines
Robert Hinch, from the University of Oxford, spoke about an intriguing new project aiming to use mathematical modelling to speed up the process of testing new vaccines. "Traditional vaccine trials take a long time," says Hinch. For the COVID-19 vaccine these trials were much quicker as the funding was available up front and the trials could run in parallel rather than sequentially. This meant the vaccine was ready to be rolled out in record time, but we are unlikely to ever get much faster than that. "To get to 100 days we need to think differently."
After a vaccine has been developed and initially tested in a laboratory, it needs to be tested using a series of clinical trials before it can be used in the population. The first two trials test that the vaccine is safe and produces the appropriate response from people's immune systems, first on a smaller group of adults (phase 1) and then on a larger group of individuals in the target group the vaccine is being designed for (phase 2). And finally a much larger scale trial tests the effectiveness of the vaccine in stopping or reducing the spread of the disease (phase 3). (You can read more about how vaccines are tested in our COVID-19 vaccine FAQ.)
"One of the reasons that phase 3 trials of the COVID-19 vaccine took as long as they did was that these began at the end of a national lockdown when there was a very low incidence of the disease," said Hinch. The PRESTO project (which stands for PREpare using Simulated Trial Optimisation) aims to make these phase 3 trials much more rapid. Computer simulations, based on mathematical models, could simulate different trial designs for different disease scenarios, to help researchers choose the most effective design of phase 3 trials. Modelling of one disease in a particular setting might suggest using a standard randomised control trial, which needs to be run over a certain length of time to ensure the trial is powerful enough to get useful results. While another scenario might suggest a ring vaccination trial (where you just vaccinate close contacts of confirmed cases) might be the better choice. "The aim is to understand key drivers across [diseases], informing which types of trials will be successful, will be the quickest and safest."
Using vaccines
Once a vaccine has been developed and tested, modelling can be used to inform decisions about how they should be used. Clapham spoke about her work in early 2021 supporting those advising the Thai government on the COVID-19 vaccination programme in Thailand. At that point vaccines were very new and there were still questions about what long term impact they would have on the spread of the disease. Different countries were in very different situations: the population in Thailand still had a relatively low exposure to the virus at that time, and the government had to decide how best to use the limited supplies of the vaccine that were available (covering less than 50% of the population).
Clapham and colleagues modelled the impact different vaccination strategies (eg. vaccinating more older people more at risk of serious consequences of COVID-19, or focussing on younger people who were more likely to spread the virus) would have on the spread of the disease. "The final decision of who to prioritise with vaccines was left to policymakers, but the research was useful for policy discussions," said Clapham.
This research is particularly interesting for its combination of specificity and generality. The research was highly specific to Thailand in early 2021, highlighting the value of tailoring models of vaccination strategies to the specific demographics of a country and their experience of a disease outbreak. But another feature of this model was that it was not for a specific COVID-19 vaccine, but covered vaccines with a range of characteristics. So while Thailand initially prioritised the over 65s for their vaccination programme, the research could continue to be used to inform the vaccination strategy as new information about the first COVID-19 vaccine, or indeed new COVID-19 vaccines, became available.
There are many other examples of modelling providing important insights throughout the design of vaccines and their use. Clapham spoke about modelling the impact of using smaller doses of a vaccine during times of shortage (for example in this paper by Joseph Wu and colleagues about using fractional doses of the Yellow Fever vaccine). "Less viral dose could give the vaccine less efficacy," says Clapham, "but it would mean more people would be vaccinated. Modelling can help understand the impact of that."
Throughout the day of talks, experts from academia, industry, and organisations such as WHO (the World Health Organisation) and the World Bank all highlighted the importance of working on these ideas now, taking what we have learned from COVID-19 and preparing for the next Disease X. "Modelling is useful," said Clapham. "But it's hard to imagine the use if it's not already there right in front of you – modelling needs to be done in advance."
About this article
This article was based on talks by Arminder Deol, Hannah Clapham and Robert Hinch at the Preventing Future Pandemics, an Open for Business event organised by the JUNIPER partnership and the Newton Gateway to Mathematics as part of the Modelling and Inference for Pandemic Preparedness programme at the Isaac Newton Institute.
Arminder Deol is Head of Data Science and Advanced Analysis at CEPI and an affiliate member of the JUNIPER partnership.
Hannah Clapham is Assistant Professor and Programme Leader of Infectious Diseases at the Saw Swee Hock School of Public Health at the National University of Singapore.
Robert Hinch is Senior Researcher in Infectious Disease Modelling at the University of Oxford's Pandemic Sciences Institute.
Rachel Thomas is Editor of Plus
This article was produced as part of our collaborations with JUNIPER (the Joint UNIversities Pandemic and Epidemiological Research network), the Isaac Newton Institute for Mathematical Sciences (INI) and the Newton Gateway to Mathematics.
JUNIPER is a collaborative network of researchers from across the UK who work at the interface between mathematical modelling, infectious disease control and public health policy. You can see more content produced with JUNIPER here.
The INI is an international research centre and our neighbour here on the University of Cambridge's maths campus. The Newton Gateway is the impact initiative of the INI, which engages with users of mathematics. You can find all the content from the collaboration here.