
Artificial intelligence algorithms are often trained on people's personal data. How can me make sure that this data remains private? This was the topic of a recent event organised by the Newton Gateway to Mathematics in collaboration with the Alan Turing Institute, called Connecting heavy tails and differential privacy in machine learning.
We talked to co-organiser Jorge González Cázares to find out more about the problem and proposed solutions. You can find out more in these three articles.
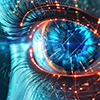
Keeping your data safe in the face of AI — The advent of artificial intelligence poses new threats to the privacy of our personal data. We explore the challenges and a way to address them.
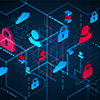
Differential privacy: Keeping your data safe — The age of Big Data poses a risk to our privacy as even anonymised data can sometimes be linked to individuals. Differential privacy provides a way of protecting sensitive information even when some of it is made public.

Maths in a minute: Stochastic gradient descent — A short introduction to an important technique in machine learning.
This article was produced as part of our collaborations with the Isaac Newton Institute for Mathematical Sciences (INI) and the Newton Gateway to Mathematics.
The INI is an international research centre and our neighbour here on the University of Cambridge's maths campus. The Newton Gateway is the impact initiative of the INI, which engages with users of mathematics. You can find all the content from the collaboration here.

