Maria Bruna is one of this year's winners of a Whitehead Prize, which is awarded annually by the London Mathematical Society to mathematicians who are in an early stage of their career. Bruna works at the University of Cambridge, the home of Plus, so we took the opportunity to talk to her to find out about her work.
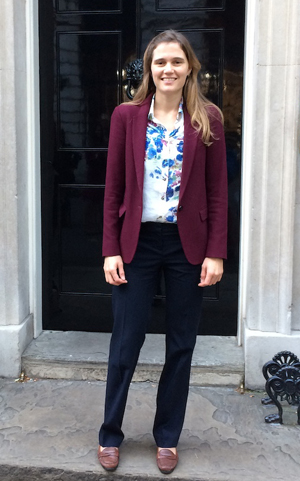
Maria Bruna.
From micro to macro
Bruna's work is about striking a balance between complexity and simplicity. When you are trying to model a system which consists of many interacting components — such as cells in the human body, dust particles, or people moving around and infecting each other with a disease — there are two obvious approaches. One is to find mathematical expressions that describe the individual components and how they interact. This gives you a highly accurate model that is also hugely complex: running it on a computer to simulate how your system will behave could take days or weeks.
The other approach is not to bother with the detail, and only describe how average quantities, such as the concentration of cells or dust particles, change over time and space. This gives you a simpler model, but you have lost the link to the microscopic behaviour. What is more, you won't know how much you have lost in accuracy by only focussing on bulk behaviour rather than individual interactions.
Bruna's work takes a third approach. She has developed systematic mathematical techniques that allow you to derive a macroscopic model, describing average quantities, from the microscopic model in all its gory detail. The macroscopic model is simpler but, since it's been derived directly from the microscopic model, it preserves key aspects of the underlying system.
"[These models] are based on partial differential equations that can describe average quantities in the system," explains Bruna. And they give you a handle on the amount of accuracy you have lost in doing the averaging. (If you have done some undergrad maths, as an analogy you can think of writing down the Taylor expansion of a complicated function: a few terms of the expansion may give you a sufficiently accurate approximation, and there's an error term to help you understand its accuracy.)
The advantage of these models isn't just that they are quicker to run on a computer, but also that they can make the workings of a system more transparent. Imagine, for example, you'd like to know how doubling the number of particles (e.g. cells or dust particles) or halving their size affects the overall behaviour of the system. A microscopic model may not offer up this information easily, so you have no choice but to run a new simulation and that could take days. "But at the macroscopic level, it's a lot easier to predict what will happen if you [change a parameter], especially because you can use all the theory of partial differential equations," says Bruna. "So you have a model that not only is much simpler to solve but also has predictive [power]."
Clearing the air
This predictive power is particularly useful when you are designing a product and want to know whether tweaking a particular feature will improve performance. One beneficiary of Bruna's expertise is the company Dyson, makers of vacuum cleaners and other gadgets that involve the flow of air. Dyson asked Bruna to help with a problem concerning the filters used in their vacuum cleaners and air purifiers: as these filters collected dust, their top layer would quickly become clogged up and stop working, so expensive filters had to be thrown away with their lower layers still clean. One way of coming up with a better filter is to try out different possibilities in the lab, but that's expensive and time-consuming.
See here to find out more about two other 2020 Whitehead Prizes.
Bruna constructed a model which used fluid mechanics to describe the flow of the air, some randomness to describe the motion of the particles of dust, and a representation of the complex geometry of the filter. The model suggested that a graded filter, whose top layer fibres are packed less densely than bottom layer ones, will last much longer. "Dyson are now also using this model to speed up their prototype production," says Bruna. "Of course this macroscopic model is not as accurate as [doing full experiments in the lab] but it allows you to see which parameters you need to look at, and once you know that, you can do more precise experiments."
From hard hats to hard maths
Bruna loves the fact that her work has direct applications in the real world. In fact, she studied industrial engineering alongside mathematics as an undergraduate in Catalonia, and thought that engineering was what she would go into. "I always saw myself with a helmet," she says. "I have also always been interested in [biology and medicine], in how we are made and how our bodies work."
What eventually lured Bruna away from engineering and towards mathematics was the versatility of maths, which she got to appreciate when doing a Masters in Mathematical Modelling and Scientific Computing at the University of Oxford. "That's when I fell in love with research. I was fascinated by how, as a mathematician, you can go from fluid mechanics, to biology, to partial differential equations."
As an applied mathematician Bruna can exploit that versatility to the full. Apart from working with Dyson, she has studied the behaviour of ant colonies and cell chemotaxis. "Especially here in Cambridge, there are so many labs and opportunities to interact and do inter-disciplinary work," she says. "That's what I am looking forward to doing more of."
Bruna only came to Cambridge a year ago, so she only had a few months to experience the old normal in this exciting new environment. "Just when I was starting to meet people, for example from the Cavendish Laboratory and the MRC Cancer Unit, lockdown struck and I was at home with my two kids," she says. "But the Whitehead Prize came at the best moment possible. It gave me the confidence to keep going."