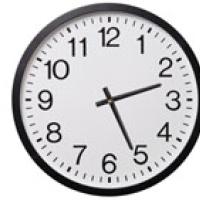
How long does it take a person infected with a virus to infect someone else? How long does it take one infection to generate another? Answering this question requires knowledge from all the levels on which a virus operates: inside our bodies, how it spreads from one person to another and how the disease behaves across the whole population. This makes the generation time of a virus both a fascinating and challenging area of research.
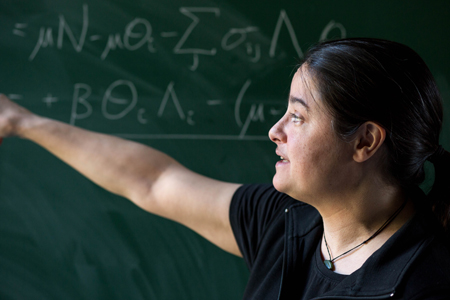
Julia Gog. Photograph by Henry Kenyon.
Understanding the generation time for COVID-19 was the topic of a virtual event held this summer by the Newton Gateway to Mathematics as part of the RAMP Continuity Network. The event was the brainchild of Julia Gog, a founding member of the JUNIPER modelling consortium. Gog set the scene with an excellent overview of the challenges and opportunities of understanding the generation time of a virus in the context of a live pandemic. (You can watch her talk online here.)
The event brought together people working in a wide variety of areas that are relevant to understanding the generation time — clinicians and researchers who study how an infection behaves within an individual (on the so-called within-host scale), scientists who try to understand how our behaviour and how our physical environment affect the spread of a disease (the between-host scale), and researchers modelling the dynamics of a disease across the whole population. "I'm very excited about this meeting," Gog said. "We [each] are experts in some of the areas, but none of us are experts in all the areas that intersect here. And bringing [this expertise] together is where some of the magic will take place!"
What is the generation time and why is it important?
The generation time isn't one single number for a particular virus. Instead it is defined on pairs of people: the infector and the infectee they go on to infect. The generation time between when one person was infected (the infector) and when they went on to infect someone else (the infectee) will be different for different pairs of people.
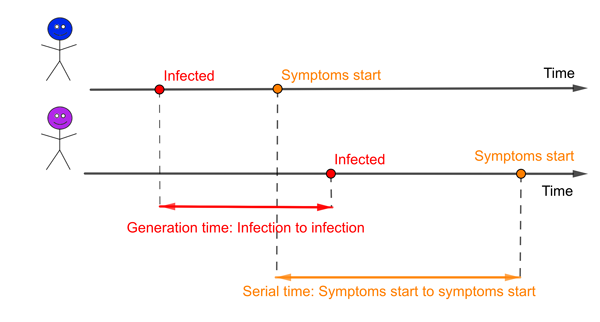
An infector (blue) and infectee (purple). The red interval represents the generation time and the orange interval the serial time.
It is also hard to measure the generation time directly. Instead it has to be inferred from things you can directly observe, such as the time interval between when the infector and the infectee develop symptoms (if they ever do — many infections are asymptomatic). So it is inevitable that assumptions need to be made about the generation time when modelling any disease.
See here for all our coverage of the COVID-19 pandemic.
Epidemiologists use statistical measures, such as the mean generation time, as parameters within their models of how the virus will behave. In particular these parameters are used in calculating the value of the reproduction ratio R, and any incorrect assumptions about the generation time can result in under- or over-estimating the value of R.
You can find out all about the generation time of COVID-19, how it is estimated and its impact on calculating R in our explanatory article Why the generation time of COVID-19 is important.
Within us, between us, all of us
The possible values of the generation time will be governed by the within-host, between-host and population-wide behaviour of the virus. In her talk at the event at the Newton Gateway, Gog highlighted some of the work that has been done at all these: "I don't think you can understand generation time in practice without thinking about all three of these."
The within-host models of how a disease behaves are not as developed as population-wide models, though some are more developed for HIV and other chronic diseases. Some simple modelling has been done using models that are similar to those used for the entire population, for example to understand the dynamics of influenza in horses. But one of the things that makes within-host modelling so hard is that it's so difficult to measure the progress of the disease. "These sorts of experiments are so hard and rare to do," says Gog. "The maths might be the same maths as population level models, but calibrating and adapting these models to observation is extremely challenging and that's one of the reasons the area is not so developed." (You can read more in Gog's paper from the Infectious Disease Dynamics 2015 programme at the Isaac Newton Institute.)
One of the key factors for understanding between-host dynamics comes from understanding the transmission routes of the disease. For COVID-19 that means understanding the airborne nature of COVID-19 and how our buildings and public spaces can influence the spread of the disease. This requires the expertise of fluid dynamicists, such as Catherine Noakes, who has played a leading role in the UK efforts to deal with COVID-19 as a participant of the Scientific Advisory Group for Emergencies (SAGE), and who was also speaking at the Gateway event. (You can read more about Noakes' work on COVID-19 in A breath of fresh air.)
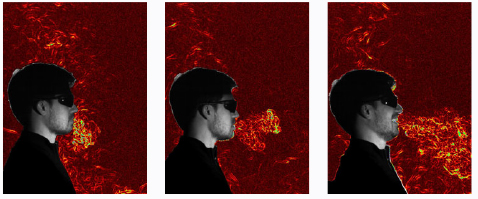
Breathing, talking and laughing without a mask. Image from Effects of ventilation on the indoor spread of COVID-19 by Bhagat, Davies Wykes, Dalziel, and Linden. Journal of Fluid Mechanics, 903, F1.
If we can link our understanding of the behaviour of the disease at these different scales, then we may be able to embed this knowledge into population-wide epidemiological models. "For me this meeting is important in itself for understanding the generation time better because it matters so much in what we're doing," says Gog, who is involved in the COVID-19 modelling work through her roles on the Scientific pandemic influenza subgroup on modelling (SPI-M) and SAGE. Gog also believes that this linking would help with understanding waning immunity and viral evolution.
"But even more exciting is getting these three scales linked together, and getting those of us with expertise in these different areas talking together. Each level on its own is not the full story, we are seeing different elements of the proverbial elephant." Events such as this one, and the work and collaboration it inspires, will hopefully give us a better picture of the current COVID-19 elephant, and any future pandemic pachyderms that cross our paths.
About this article
This article is based on Julia Gog's talk at the Understanding the generation time for COVID-19 event that took place at the Newton Gateway for Mathematics in July 2021.
Gog is a founding member of the JUNIPER modelling consortium, and a member of SPI-M, a modelling group which feeds its results into the Scientific Advisory Group for Emergencies (SAGE), as well as of the steering committee of a national consortium, led by the Royal Society, to deal with the COVID-19 pandemic.
Rachel Thomas is Editor of Plus.
This article was produced as part of our collaborations with JUNIPER, the Joint UNIversity Pandemic and Epidemic Response modelling consortium, and the Isaac Newton Institute for Mathematical Sciences (INI).
JUNIPER comprises academics from the universities of Cambridge, Warwick, Bristol, Exeter, Oxford, Manchester, and Lancaster, who are using a range of mathematical and statistical techniques to address pressing question about the control of COVID-19. You can see more content produced with JUNIPER here.
The INI is an international research centre and our neighbour here on the University of Cambridge's maths campus. It attracts leading mathematical scientists from all over the world, and is open to all. Visit www.newton.ac.uk to find out more.
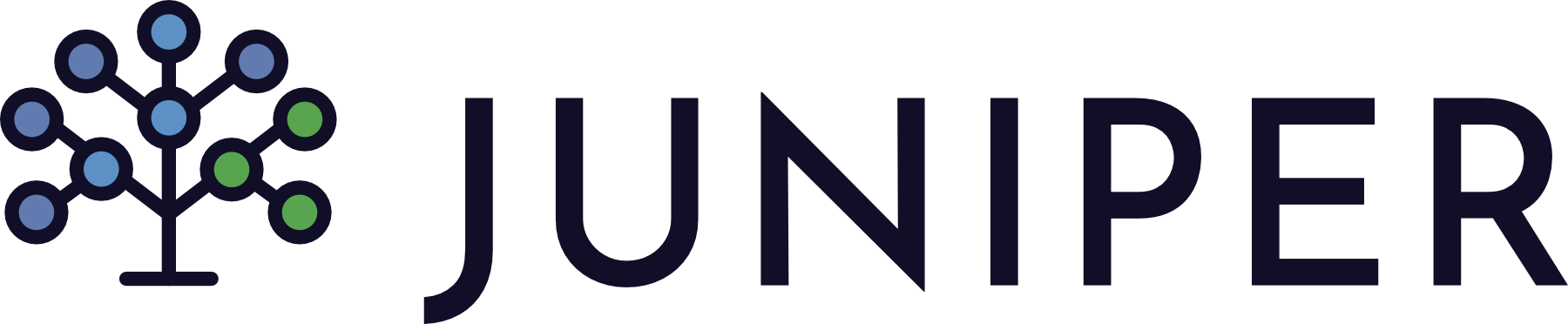
