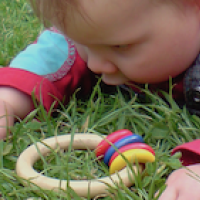
You are reading this article. Have you ever thought about how you are doing that? How is your brain interpreting what your eyes see on the screen: recognising the shapes as letters, recognising the groups of letters as words and the groups of words as sentences? How is your brain translating these words and sentences into thoughts that, hopefully, convey these questions that I am proposing to you?
This process of reading and engaging with the previous paragraph and imagining how your brain might work, might seem a straightforward task to you. For a machine, parts of this process are already possible: text recognition software already exists that identifies the text in an image as a particular language, indeed the Google Translate app will even translate a screenshot of the previous paragraph into German, Japanese, Welsh or many other language. But we have not yet managed to build a machine that is also able to contemplate the meaning of these sentences and imagine how our brains might work (without resorting to an internet search) – a true artificial intelligence does not yet exist.
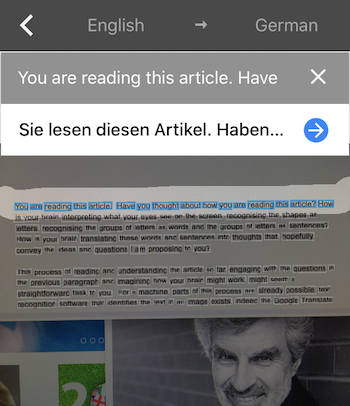
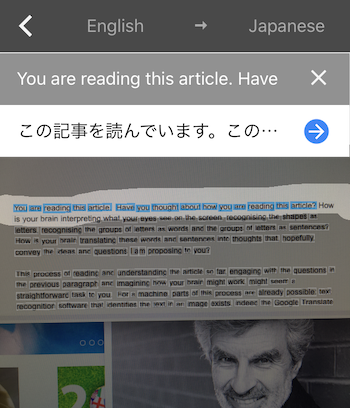
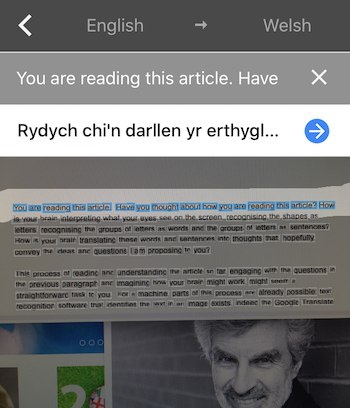
Artificial intelligence in action: Screenshots of this article, submitted to Google Translate. It identifies the text in the image as English and translates it to a wide variety of languages.
Acting to understand
Yoshua Bengio, Professor at the University of Montreal and winner of the 2018 Turing Award, describes tasks such as recognising and reading the words on this screen as System 1 tasks: those that are intuitive, fast and essentially unconscious. In contrast, System 2 tasks are slower, require conscious effort, and involve reasoning and thinking in a logical way: for example, understanding the meaning of sentences, or imagining the process that allows our brains to comprehend them. Bengio believes that a true artificial intelligence should be able to do both of these system 1 and system 2 types of cognitive tasks.
So what do we, humans, have that makes us so good at system 2 tasks, that machines lack? Bengio believes the answer to that question is agency: our ability to interact with the world, influence our environment and observe and adapt to results of our actions. This agency is the key to our ability to build an internal model of how the world works.
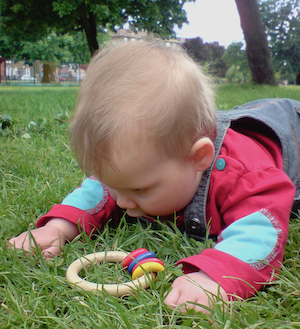
Physicist at work... (Photo: Rachel Thomas)
We start building such models of the world as children. I remember my baby son's fascination as he pushed things, picked things up, dropped them, and threw them. As he interacted with his environment he learnt about cause and effect, what would happen next. A favourite game was to throw everything out of his high chair or pram. Not only did he learn that his sleep deprived parents invariably retrieved his toys, spoons, and cups for him, but he also learnt about gravity. As he learnt to walk he began to learn about his centre of mass. "A two-year-old knows intuitive physics not with equations, not taught by their parents. They experiment and see what happens," says Bengio. "I think that in order to make progress towards machines that understand the world, to get closer to human level intelligence, we need machines to interact with the world, just like babies."
The agent perspective
This ability to build understanding by interacting with the world is what Bengio calls the agent perspective. For a machine to do this, it wouldn't necessarily need bodies like ours to interact with the world. In the first instance a machine interacting by text, or verbally, with a person, is a way to interact with their environment.
Bengio is a leading expert on artificial intelligence, working on machine learning. This involves machines learning how to do a specific task themselves, rather than being explicitly taught how to do it by human programmers. The machines are given data sets, so-called training data, to learn from (find out more in this introduction to machine learning).
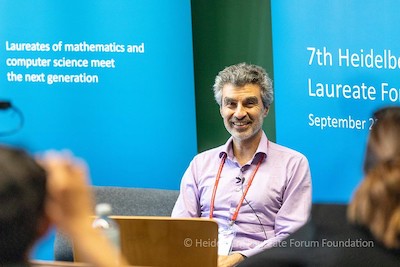
Yoshua Bengio (Photo copyright: Heidelberg Laureate Forum Foundation)
Much of the theory of machine learning has been based on the assumption that the data sets a machine will learn from will be fixed - there will always be the same distribution of data. "We train the machine on data in the lab, on data from a particular country. But when we deploy machine learning for real [in the word], there will be a change in the distribution of the data as the world changes," says Bengio.
For example, the machine may start working with data from a new country. Bengio suggests we consider what happens when you move from a country where one drives on the right to one where you are supposed to drive on the left. You can learn and remember the new rules, and adapt your behaviour to this new situation (a system 2 ability). Then with a lot of conscious attention and months of practice it will become a habit and you won't have to concentrate on driving on the correct side of the road anymore – it will become a system 1 task. This ability to flexibly adapt and reason your way into new situations (system 2 abilities) and then gradually convert this into practiced skills (system 1 abilities) is something which we would like machines to also be able to do. For this to be possible your brain quickly adapts to new situations by reusing the pieces of knowledge you already have, in rather novel ways.
"The agent perspective means that the learner can do things in the world, they can push things around, and this can have consequences." When agents are doing things in the world it changes the distribution of the data they are working with. When we spoke to Bengio at the Heidelberg Laureate Forum in September 2019, he had unfortunately broken his foot just the day before. "Yesterday I had a little accident and now the signals that come to my brain from my body are very different. I have to learn to walk differently [on crutches]. My brain can adapt very quickly to these things. This ability [of a machine] to adapt to changes in the world due to interventions by agents would be very useful in practice, it's not just of theoretical concern."
Finding a way for machines to interact with their environment and adapt to the changes in the data they receive might finally allow machines to have a deeper causal understanding of the world they exist in. As we'll see in the next article.
About this article
Rachel Thomas is Editor of Plus. She spoke to Yoshua Bengio at the Heidelberg Laureate Forum in September 2019.
This article now forms part of our coverage of a major research programme on deep learning held at the Isaac Newton Institute for Mathematical Sciences (INI) in Cambridge. The INI is an international research centre and our neighbour here on the University of Cambridge's maths campus. It attracts leading mathematical scientists from all over the world, and is open to all. Visit www.newton.ac.uk to find out more.

Comments
Ageticity
So, can there be an algorithm for agenticity?