
The growth rate of a disease is a natural way to capture how quickly the number of new infections are changing day by day. The growth of cases of a disease is modelled using an exponential curve:
Here
For example, the growth rate for HIV is
If the growth rate is positive, the number of new cases each day is increasing. And if the growth rate is 0, the number of new cases stays constant. What is needed to keep an epidemic under control is for the growth rate to be negative and hence the number of new cases to be decreasing.
But what does the value of the number actually mean? If the number of new cases has decreased by 3% since yesterday, then the growth rate is, approximately,
However, for larger values of lambda (eg
Why isn't R enough?
If you have already studied a bit of epidemiology, you have probably come across the concept of R, the reproduction ratio (or sometimes called the reproduction number) of a disease. That's the number of people infected, on average, by a single infected person. As explained here, R helps us understand what is happening with the disease: R>1 means that the epidemic will grow, R=1 means we are plateauing, R1 means that the epidemic will decline.
One thing that R does not tell us, though, is how quickly things are changing. This is because R is not a rate, there is no timescale involved. For example, if R=2 for some disease then we know the epidemic will grow (because R>1), but we cannot tell how quickly. For diseases like HIV or TB, where there can be months or years between one person infecting the next person, even R=2 means slow growth over time. However for influenza or measles, where the infection is much faster, on the scale of days, R=2 means very rapid growth.
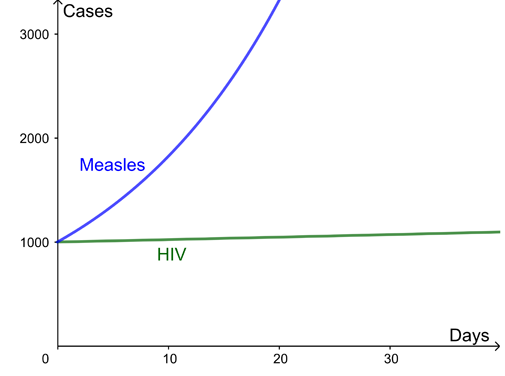
Here are two example curves for the growth of infection, both with a reproduction ratio R=2. The difference is the time between new infections – several months for HIV but just days for measles.
Which is better: R or the growth rate?
Both the reproduction ratio
Reproduction ratio: R | Growth rate per day: |
---|---|
R is more natural for understanding strength of the intervention needed to stop an epidemic, better for planning control measures. For example:
|
Growth rate is more natural for thinking about how cases change over time. For example
|
R>1 exponential growth R=1 flat R1 exponential decay |
|
R a ratio of cases by infection generation. It is not a rate: there is no timescale involved. |
The growth rate |
R is not at all easy to measure in practice, but can be fitted using models if the timescales of infection are known. In principle it could be estimated by detailed epidemiological data on exactly who got infection from whom, but this is not usually feasible in typical settings. |
The growth rate |
Both the reproduction ratio and the growth rate are particularly hard to estimate when the number of cases is small, for example if the incidence of the disease is very low, or if the community you are studying has a very small population. In that case, day to day fluctuations can easily swamp the underlying patterns of the disease, so you will have greater uncertainty about the growth rate (and so expect wider confidence intervals).
How do you get from R to the growth rate and vice versa?
The precise relationship between R and the growth rate is not straightforward: it needs to take into account the timings of each infection to the next. A crude approximation is
where
I can cope with some advanced maths, tell me more!
OK! All of this supposes that the control measures and number of people susceptible to the disease are not changing too quickly.
Following one infected person, denote their time since infection by
and yes, this is very closely related to a Laplace transform, or a moment generating function for the generation time distribution.
For specific distributions for the generation time, for example a gamma distribution, this can sometimes be simplified. Taking the generation time to be exactly constant, say
See this paper by Wallinga and Lipsitch for more details.
About this article
Rachel Thomas and Marianne Freiberger are the Editors of Plus. This article was produced with Julia Gog, Professor of Mathematical Biology at the University of Cambridge and co-lead of the JUNIPER network. Gog is also a member of the Scientific Pandemic Infections group on Modelling (SPI-M), an advisory group of the Department of Health and Social Care which provides expert advice to the UK government based on infectious disease modelling and epidemiology and fed results into the Scientific Advisory Group for Emergencies (SAGE) during the COVID-19 pandemic.
This article is part of our collaboration with JUNIPER, the Joint UNIversities Pandemic and Epidemiological Research network. JUNIPER is a collaborative network of researchers from across the UK who work at the interface between mathematical modelling, infectious disease control and public health policy. You can see more content produced with JUNIPER here.
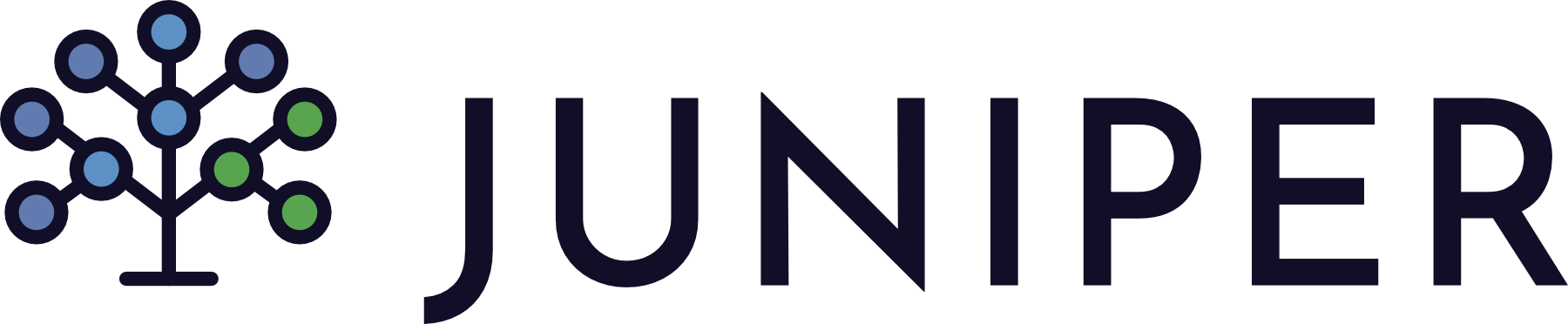