To control the COVID-19 pandemic, and keep the death toll as low as possible, it's essential to quickly diagnose people who have the disease. This is done using lab tests, but no test is 100% accurate. The COVID-19 test has a relatively high rate of false negatives (30%), which indicate a patient is clear when in reality they have the disease, and lead to late diagnosis and treatment. This doesn't only produce worse outcomes for patients, but also means they can spread the disease further when they are released from hospital.
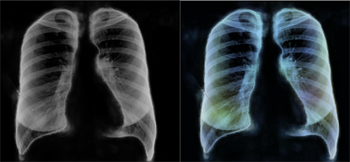
Chest X-ray images which have been pre-processed so an AI algorithm finds them easier to analyse.
When the test does correctly diagnose a patient with the disease, a correct prediction of whether they will need to go to hospital — and if yes, whether they will need intensive care or intensive ventilation — is essential in order to efficiently allocate medical resources.
A collaboration between mathematicians, clinicians and researchers from around the world is proposing that artificial intelligence might be able to help in this context. The AIX-COVNET project, led by Carola-Bibiane Schönlieb, Professor of Applied Mathematics, and Evis Sala, Professor of Oncological Imagin, both from the University of Cambridge, aims to develop an artificial intelligence tool that can accurately diagnose a patient with COVID-19 from clinical and lab data, along with chest X-ray and CT images. The test will also provide support for patient triaging and prognosis, predict the likely clinical outcomes for the individual, and help clinicians to plan their treatment and manage hospital resources.
The approach
The tool will be based on machine learning. This involves algorithms learning to spot patterns in data sets (e.g. CT scans of patients' chests) that are connected to a particular outcome (e.g. the patient has COVID-19). The learning process happens on so-called training data for which (in the case of supervised learning) the correct answer is known (e.g. whether a patient has COVID-19). While sifting through the training data the algorithm will tune the values of parameters that have been built into it, until it can reliably spot patterns connected to the correct outcome and give the correct answer.
See here for all our coverage of the COVID-19 pandemic.
Simple machine learning algorithms are surprisingly straight-forward (see this article to find out more), but the field has now become incredibly sophisticated. A form of machine learning known as deep learning can learn without human supervision from unstructured and unlabelled data sets. The AIX-COVNET tool will utilise deep learning approaches combined with rigorous mathematical and statistical techniques that are customised to the needs of COVID-19.
Many challenges
While deep learning techniques are well-developed, applying them to COVID-19 presents a range of challenges. One is that useful data to be used for training is scarce. "Few data sets are publicly available and almost all the data comes from a few sources, mainly China," says Schönlieb. "So data might not represent the global population."
Another question is how useful medical images, in particular X-rays, can be in diagnosing COVID-19. A recent study suggests that COVID-19 may not leave enough of a fingerprint on X-ray images for an algorithm to distinguish it from other forms of viral pneumonia. CT scans appear to fare better, but since these are not as commonly used in many countries as X-rays, this potential limitation needs to be addressed.
There are also challenges regarding the ambitious goal to not just diagnose COVID-19, but also predict the development of the disease in a patient. At the moment it is not entirely clear what kind of factors are important in prognosis and prediction, so it's not clear what kind of data to feed to an algorithm. There are interesting possibilities here: while most existing work focusses on the lungs, the heart is also a potentially important organ for prognosis.
A promising start
Since the AIX-COVNET project started on March 23, 2020 the team have been exploring ways of overcoming various challenges. They have proposed a machine learning framework for integrating data from very different sources, such as lab results and images, and looked into how to address the problem of data sets being incomplete. They have been looking into how one might help an algorithm to spot patterns in lung tissue and are also developing algorithms to spot patterns in images of the heart to signify complications that may arise in COVID-19 patients.
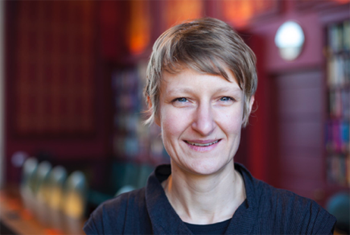
Carola-Bibiane Schönlieb.
A particularly interesting problem the AIX-COVNET team have been looking at comes from the fact that machine learning algorithms are "lazy" and will "cheat" whenever they can. For example, imagine an algorithm learning from training data what kind of patterns in chest X-ray images are connected to COVID-19. If much of the training data comes from a single hospital that has treated a lot of COVID-19 cases, then the algorithm will learn easy-to-spot patterns connected to that hospital and link those to COVID-19, rather than learning clinically relevant patterns.
For example, it may learn the font the hospital uses to label X-ray images with patients' names, and decide that whenever an image has that font in its labelling, the corresponding patient has COVID-19. One way of getting around this problem is to pre-process X-ray images so that potentially confounding patterns are removed. The AIX-COVNET team have been exploring viable techniques for doing this.
The results achieved so far are promising, but much remains to be done. "These are preliminary results of an active collaboration that is working on various [aspects] of the AIX-COVNET analysis vision," says Schönlieb. She and her team are inviting researchers and clinicians from around the world to contribute to the collaboration, so that a fully functioning AI tool is available for use as soon as possible.
"The strength of our team is the close dialogue between clinicians and data scientists and the passion we all bring to advancing AI solutions for COVID-19," she says. While the end goal may seem ambitious, the willingness of so many people from around the world to voluntarily give up their time, resources and expertise gives grounds for optimism.
You can find out more about the AIX-COVNET project on its website and in this video featuring Carola-Bibiane Schönlieb.
This article now forms part of our coverage of a major research programme on deep learning held at the Isaac Newton Institute for Mathematical Sciences (INI) in Cambridge. The INI is an international research centre and our neighbour here on the University of Cambridge's maths campus. It attracts leading mathematical scientists from all over the world, and is open to all. Visit www.newton.ac.uk to find out more.
