
In the first part of this article we looked at a psychological study which claims to provide evidence that certain types of extra-sensory perception exist, using a statistical method called significance testing. But do the results of the study really justify this conclusion?
What's wrong with p values?
Despite the very widespread use of statistical significance testing (particularly in psychology) the method has been heavily criticised, by psychologists themselves as well as by some statisticians and other scientists.
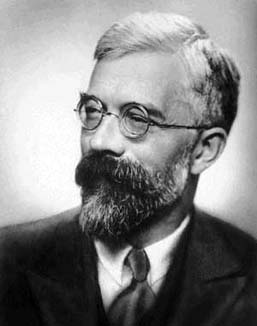
R.A. Fisher invented the notion of significance testing.
One criticism that is relevant here concerns alternatives to the null hypothesis. Remember the conclusion that was reached when the p value in Bem's experiment was 0.009? "Either the null hypothesis really is true, but nevertheless an unlikely event has occurred. Or the null hypothesis isn't true." This says nothing about how likely the data were if the null hypothesis isn't true. Maybe they are still unlikely even if the null hypothesis is false. Surely we can't just throw out the null hypothesis without further investigation of what the probabilities are if the null hypothesis is false?
This is an issue that must be dealt with if one is trying to use the test to decide whether the null hypothesis is true or false. It should be said that the great statistician and geneticist R.A. Fisher, who invented the notion of significance testing, would simply not have used the result of a significance test on its own to decide whether a null hypothesis is true or false — he would have taken other relevant circumstances into account. But unfortunately not every user of significance tests follows Fisher's approach.
The usual way to deal with the situation where the null hypothesis might be false is to define a so-called alternative hypothesis. In the case of the Bem experiment this would be the hypothesis that the average rate of correct answers is greater than 50%. You might think that we could just calculate the probability of getting the Bem's data on the assumption that the alternative hypothesis is true. But there's a snag. The alternative hypothesis simply says that the average rate is more than 50%. It doesn't say how much more than 50%. If the real average rate were, let's say, 99%, then getting an observed rate of 51.7% isn't very likely, but if the real average rate were 51.5%, then getting an observed rate of 51.7% is quite likely. But real averages of 99% and of 51.5% are both covered by the alternative hypothesis. So this isn't going to get us off the hook.
Let's do Bayes
One possibility is to meet the issue of misinterpreting the p value head on. I said that many people think that a p value of 0.009 actually means that the probability that the null hypothesis is true is 0.009, given the data that were observed. Well, I explained why that's not correct — but why do people interpret it that way? In my view it's because what people actually want to know is how likely it is that the null hypothesis is true, given the data that were observed. The p value does not tell them this, so they just act as if it did.
The p value does not tell people what they want to know because in order to find the probability that the null hypothesis is true (given the data), one needs to take a Bayesian approach to statistics. There's more than one way to do that, but the one I'll describe is as follows.
It uses the odds form of Bayes' theorem, the theorem behind Bayesian approaches to statistics. There's much more on this in our articles How psychic was Paul?, The logic of drug testing and It's a match.
Let's writeSo far, so good. But to find the posterior odds for the alternative hypothesis, we need to know the prior odds for the alternative hypothesis as well as the Bayes factor. It is the existence of the prior odds in the formula that puts some people off the Bayesian approach entirely.
The prior odds is a ratio of probabilities of hypotheses before the data have been taken into account. That is, it is supposed to reflect the beliefs of the person making the calculation before they saw any of the data, and people's beliefs differ in a subjective way. One person may simply not believe it possible at all that ESP exists. In that case they would say, before any data were collected, that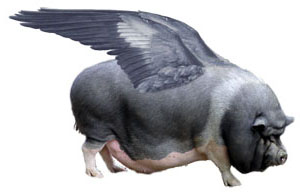
Hey look! A flying pig! People have different prior odds for the existence of ESP.
Another person might think it is very unlikely indeed that ESP exists but not want to rule it out as being absolutely impossible. This may lead them to set the prior odds for the alternative hypothesis, not as zero, but as some very small number, say 1/10,000. If the Bayes factor turned out to be big enough, the posterior odds for the alternative hypothesis might nevertheless be a reasonably sized number so that Bayes' theorem is telling this person that, after the experiment, they should consider the alternative hypothesis to be reasonably likely.
Thus different people can look at the same data and come to different conclusions about how likely it is that the null hypothesis (or the alternative hypothesis) is true. Also, the probability that the null hypothesis is true might or might not be similar to the p value — it all depends on the prior odds as well as on the Bayes factor. (In many cases, actually it turns out that the probability of the null hypothesis is very different from the p value for a wide range of plausible values of the prior odds, drawing attention yet again to the importance of being pedantic when saying what probability is described by the p value. This phenomenon is sometimes called Lindley's paradox, after the renowned Bayesian statistician Dennis Lindley who drew attention to it.)
The Bayes factor
You might think that the issue of people having different prior odds could be avoided by concentrating on the Bayes factor. If I could tell you the Bayes factor for one of Bem's experiments, you could decide what your prior odds were and multiply them by the Bayes factor to give your own posterior odds.
In fact, one of Bem's critics, Eric-Jan Wagenmakers (see here for a paper criticising Bem's work), takes exactly that line, concentrating on the Bayes factors. For Bem's Experiment 2, for instance, Wagenmakers and colleagues calculate the Bayes factor as about 1.05. Therefore the posterior odds for the alternative hypothesis are not much larger than the prior odds, or putting it another way, they would say that the data provide very little information to change one's prior views. They therefore conclude that this experiment provides rather little evidence that ESP exists, and certainly not enough to overturn the established laws of physics. They come to similar conclusions about several more of Bem's experiments and for others they calculate the Bayes factor as being less than 1. In these cases the posterior odds for the alternative hypothesis will be smaller than the prior odds; that is, one should believe less in ESP after seeing the data than one did beforehand.
Well, that's an end of it, isn't it? Despite all Bem's significance tests, the evidence provided by his experiments for the existence of ESP is either weak or non-existent.
But no, it's not quite as simple as that. The trouble is that there's more than one way of calculating a Bayes factor. Remember that the Bayes factor is defined asWagenmakers and his colleagues used one standard method of calculating the Bayes factors for Bem's experiment, but this makes assumptions that Bem (with Utts and Johnson) disagrees with. For Experiment 2 (where Wagenmakers and colleagues found a Bayes factor of 1.05) Bem, Utts and Johnson calculate Bayes factors by four alternative methods, all of which they claim to be more appropriate than that of Wagenmakers and they result in Bayes factors ranging from 2.04 to 6.09.
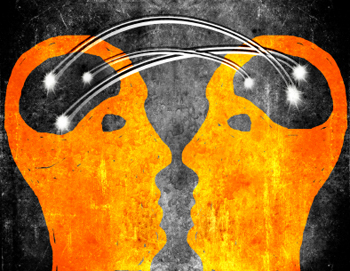
Can experimental data ever provide strong evidence for ESP?
They calculate Bayes factors around 2 when they make what they describe as "sceptical" prior assumptions, that is, assumptions which they feel would be made by a person who is sceptical about the possibility of ESP, though evidently not quite as sceptical as Wagenmakers. The Bayes factor of about 6 comes from assumptions that Bem and his colleagues regard as being based on appropriate prior knowledge about the size of effect typically observed in psychological experiments of this general nature.
These Bayes factors indicate considerably greater evidence in favour of ESP, from the same data, that Wagenmakers considered to be the case. Bem, Utts and Johnson report similar results for the other experiments.
Since then Wagenmakers has come back with further arguments as to why his original approach is better (and as to why Bem, Utts and Johnson's "knowledge-based" assumptions aren't based on the most appropriate knowledge). Who is right?
Well, in my view that's the wrong question. It would be very nice if experiments like these could clearly and objectively establish, one way or the other, whether ESP can exist. But the hope that the data can speak for themselves in an unambiguous way is in vain. If there is really some kind of ESP effect, it is not large (otherwise it would have been discovered years ago). So any such effect must be relatively small and thus not straightforward to observe against the inevitable variability between individual experimental participants. Since the data are therefore not going to provide really overwhelming evidence one way or the other, it seems to me that people will inevitably end up believing different things in the light of the evidence, depending on what else they know and to some extent on their views of the world. Just looking at the numbers from Bem's experiments is not going to make this subjective element disappear.
About the author
Kevin McConway is Professor of Applied Statistics at the Open University. As well as teaching statistics and health science, and researching the statistics of ecology and evolution and in decision making, he works in promoting public engagement in statistics and probability. Kevin is an occasional contributor to the Understanding Uncertainty website.
Comments
Overcomplicating the issue
It may be obfuscating the issue to suggest that p-values alone are not strongly suggestive of some genuine effect in an experiment like this. The reason is that unless the experiment is flawed, the only probability distribution of results that can occur without an interesting effect is that of the null hypothesis. Of course a single p-value like 0.009 is not by itself indicative of anything but a fluke: run 100 experiments and this sort of result is rather likely. This is why the particle physics community demands p-values of 0.0000003 before using the term "discovery". I don't believe they qualify this with a Bayesian criterion: the null hypothesis is simply the hypothesis that the hypothesis is not true.
It is perhaps inaccurate to say that no effect has been discovered years ago: for example, the book "Entangled Minds" by Dean Radin (2006) presents meta-analysis of a wide range of possible effects from experiments over several decades and finds qualitatively similar weak effects for most of them. The p-values are much lower in some cases, with much larger effective sample sizes. If I understand the data correctly, the effects cannot be adequately explained by the hypothesis that some of the experiments are flawed or fraudulent, because the distribution of the results of different experiments is much more like a weak effect with the variation in the results being the result of sampling. I would welcome the views of an expert like McConway on Radin's claims.
So it may be more accurate to say that (1) these effects have not been accepted as definitely genuine by mainstream psychology (or other sciences) and (2) there is little or no understanding of mechanisms that might explain the effects.
I feel it is this lack of any real scientific understanding of the nature of the purported phenomenon that blocks acceptance rather than the statistics themselves. Weaker statistics have been used (rightly in my opinion) to make major strategic decisions in other fields. Given a strong p-value (say 0.000001) in an experiment, two intelligent people can come to two different conclusions. The first might say "unless this experiment is faulty or fraudulent, there is very likely a real effect here". The second might say "this experiment is almost certainly faulty or fraudulent, since the conclusion is ridiculous". If ESP effects are weak in the way that Radin's analysis and this experiment (and many others) suggest, it would take a rather large experiment (or a genuine effect plus a bit of luck) to even reach these sorts of p-values, though more extreme ones can be found by combining data from many different experiments.
Liam Roche