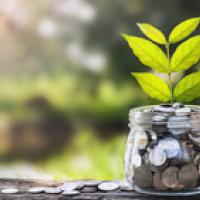
Brief summary
A mathematical model is a description of a process in the world around us that is written in terms of mathematical expressions. You've probably intuitively used mathematical models without even realising it, such as calculating how long it will take you to save £100 if you save £10 a week.
Mathematical models are always simplifications of reality and involve uncertainties, but a good model can provide the most educated guess we're ever going to be able to make about how a process may play out.
A mathematical model is a description of a process in the world around us in terms of mathematical expressions. You can use the model to get an idea of how the process may play out, or how this might change if circumstances change.
You have probably used a simple mathematical model without even realising it. Imagine, for example, you have opened a bank account and are planning to pay in
Writing
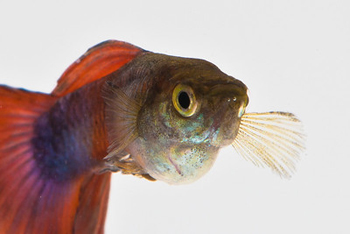
Mathematical models can help you understand the growth of animal populations. Photo: lens-flare.de, CC BY-NC 2.0.
Our example shows how powerful mathematical models can be. Once you've decided how much to save each month the formula above gives you full knowledge of your savings into the distant future. It also allows you to explore different scenarios by changing the value of the parameter.
But the example also highlights some of the limitations of mathematical modelling. It hinges on the assumption that you'll pay in the same amount, unfailingly, every month. But life is rarely that predictable. To get useful predictions from your model, you need to think hard about what value to choose for the parameter
The model also assumes that you're not earning any interest on your savings — however, any decent savings account will pay you some interest. To take account of this, the mathematical expression in the model needs to be more complex (see here to find out more). Alternatively, you may stick with the simpler formula just because it is easier to use. If you do that you need to be aware of the inaccuracy that creeps into the predictions as a result of your approximation (it's by not taking account of interest accrued on debt that people sometimes find themselves owing a lot more money than they were expecting).
Mathematical models are used in a huge range of different areas. They are essential in engineering, for example to see how air will flow around a car or a plane. They are used to forecast the weather and the Earth's climate. They can also be used to study how animal populations grow, and to see how fast an infectious disease might spread. The areas of application of mathematical models are nearly endless.
Although the basic idea behind any mathematical model is as simple as in our example, the models themselves can be a lot more complex. The mathematical expressions involved can be far more complicated (for example they can consist of one or more differential equations) and sometimes the calculations are so difficult, computers need to be used to find approximate answers. Models can also involve many parameters, and the values these parameters should take may not be known in advance. In that case, the values need to be estimated from real-life observations of the process you're modelling. Whatever equations and parameters you come up with, unless you are dealing with an extremely basic process, the model is only ever going to be a simplification of reality.
Despite these limitations, mathematical models can be extremely powerful. Some are so reliably accurate, we can base important decisions on them. Others involve more uncertainty, but they still allow us to explore general trends and see how things would change if important parameters change. Being aware of limitations is key. When dealing with a mathematical model it's essential to understand the assumptions it is based on, and the uncertainties that can creep in, for example when approximations are used or when the values of parameters are estimated (or when you are dealing with a chaotic system). There are mathematical and statistical techniques to help getting to grips with some of these uncertainties (indeed, uncertainty quantification is an area of maths in its own right). If you can, you should always compare the predictions of a model with reality, to see whether they are accurate, before using the model for practical purposes.
In summary, mathematical models are always simplifications of reality and involve uncertainties, but a good model can provide the most educated guess we're ever going to be able to make about how a process may play out. To quote the (rather understated) observation of the statistician George Box, "All models are wrong, but some are useful."
To see all our content involving mathematical models, click here.
This article is part of our collaboration with JUNIPER, the Joint UNIversities Pandemic and Epidemiological Research network. JUNIPER is a collaborative network of researchers from across the UK who work at the interface between mathematical modelling, infectious disease control and public health policy. You can see more content produced with JUNIPER here.
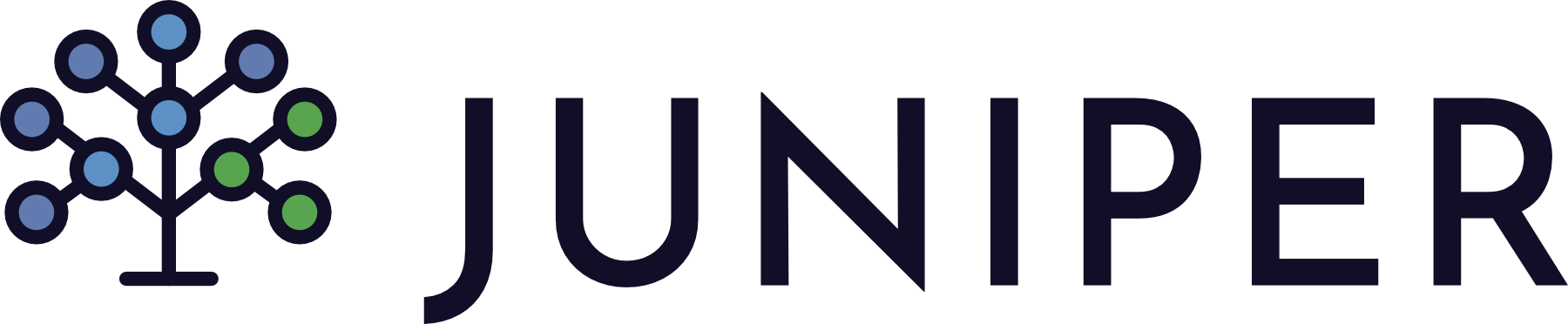
This article forms part of our collaboration with the Isaac Newton Institute for Mathematical Sciences (INI) – you can find all the content from the collaboration here.
The INI is an international research centre and our neighbour here on the University of Cambridge's maths campus. It attracts leading mathematical scientists from all over the world, and is open to all. Visit www.newton.ac.uk to find out more.
