
See here for all our coverage of the COVID-19 pandemic.
It's often assumed that in an emergency people will panic until the emergency services appear, from which point onwards they will calm down and do as they are told. "But we have overwhelming evidence to suggest that people become inter-dependent and cooperative. Panic does exist, but it is rare."
Brooke Rogers is a behavioural scientist at King's College, London, who has extensively studied people's responses to dangerous situations. The evidence she quotes here comes from studies into disasters such as 9/11, the London 7/7 tube bombings, and many other emergencies. It shows that when it comes to planning for, or dealing with, an extreme event, insights from behavioural science are crucial. If you don't understand how people will behave, your plans and measures are bound to fail.
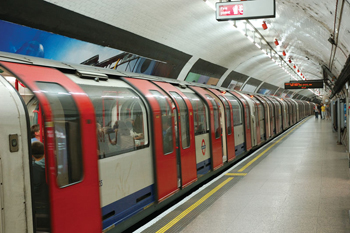
Studies of disasters like the London 7/7 tube bombings show that panic is rare. Photo: CornyTheEditor, CC BY-SA 4.0.
This is true of the COVID-19 pandemic as much as it is for terrorists attacks or natural disasters, which is why Rogers was a main speaker at a recent event hosted by the Newton Gateway for Mathematics. The aim was to encourage and enable communication between epidemiological modellers — those who focus on the maths of a pandemic to understand the spread of disease in the population — and behavioural scientists.
"Public behaviour impacts the dynamics of an infectious disease outbreak," says Ed Hill, an epidemiologist of the University of Warwick and member of the JUNIPER modelling consortium, who co-organised the event. "Who we make contact with, what interventions we make use of — social distancing, face coverings, testing, isolating if have COVID-like symptoms — whether we have been vaccinated, etc, all impact the course of the disease," he explains.
"Also, we ultimately want to minimise the collective harms of interventions such as lockdowns as much as possible. To do that, we need to encourage conversations between epidemiologists and public health modellers, and enable epidemiologists to engage with those who model behaviours, the people who design policies, as well as with economic epidemiologists."
This was the aim of the Newton Gateway event, which was guided by links with the JUNIPER consortium and delivered by the RAMP Continuity Network.
Maths and people
At first sight it's hard to see how something as complex, and fickle, as human behaviour can be built into the numbers game that is epidemiological modelling. But the fact is that one aspect of it is already part of all respectable models. They are based on information about people's contact patterns (see this article to find out more). The information comes from surveys that asked people about their contacts in a typical day. Such surveys, and the insights they delivered, featured strongly at the Newton Gateway event.
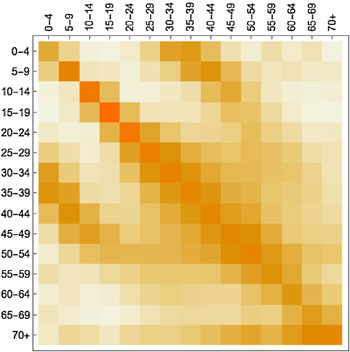
This is a contact matrix, which displays average contacts between different age groups. Darker shades indicate more contacts. Didsease models make use of such contact data. The figure is from the paper Contagion! The BBC Four Pandemic – The model behind the documentary. Used by permission.
People's behaviour also appears in epidemiological models in other ways. The recent projections of the so-called Warwick model, which informs government policy through the Scientific Pandemic Influenza Group on Modelling (SPI-M), includes a parameter designed to reflect people's level of precaution about catching COVID-19.
In their most recent report the JUNIPER researchers behind the model ran simulations for seven different scenarios for how that parameter might change in the future, giving a sense of how people's behaviour might influence the number of COVID-19 cases over autumn and winter (see this article to find out more).
This approach already delivers revealing insights, but the scenarios are educated guesses applied in a blanket fashion across all of society. This, says Hill, could and should be improved. "Considering everyone in the population to have the same behaviour in response to public health interventions may make results from disease outbreak models less reliable."
To comply or not comply?
Caroline Walters, an epidemiologist of Imperial College, London, has developed a blueprint for a more nuanced approach to capturing human behaviour. Walters used a psychological theory that applies in situations where there's a threat to people's health and a measure that helps reduce it, such as wearing a mask or using hand sanitiser. Put briefly, Extended parallel processing says that whether or not people will comply with the suggestion to use the measure depends on two things: how high they perceive the threat to their health to be and to what extent they think the measure actually works.
This makes intuitive sense, and the beauty is that you can express it in mathematical form. Quantifying perceived threat and perceived efficacy of the measure by numbers, you can then come up with a mathematical function that expresses the probability a person will comply (wear a mask or use sanitiser) as a function of the perceived risk and efficacy. The nature of this function should of course be informed by psychological insights (see the box below for an example).
Once you have the probability of compliance you can integrate it into an epidemiological model. Walters, together with colleagues, has done just that and she presented this work at the Newton Gateway event. It involves a toy model which explores where a hand sanitiser should ideally be placed at airport security to reduce the probability of infection, which depends, among other things, on the probability that people comply and use the sanitiser when they see it (see Walters' paper for details).
Modelling compliance
Write
The results show that people’s attitudes can matter: the optimal location of the hand sanitiser, according to the model, doesn’t just depend on how much protection it gives from infection, but also on the exact combination of values of perceived threat and perceived efficacy.
It's just a toy model not designed to be used in practice, but it's an important proof of concept. It shows, not only how a psychological theory might be integrated into an epidemiological model, but also that doing so makes sense. If people's perceptions can make a real difference to the outcome of a model then they should be taken into account.
Quantifying those perceptions is difficult of course, especially since they will vary over different groups. But getting definite answers is rarely the aim of modelling. Instead, an approach like Walters' might help researchers identify what kind of attitudes are particularly useful, or particularly damaging. Behavioural scientists can then use this information to design the messages the government puts out to the public.
Protecting livelihoods
One of the great tragedies of the COVID-19 pandemic is that it seriously impacted many people's livelihoods. So far, the epidemiological models that inform government policy have focussed on the direct health harms of COVID-19: infections, hospitalisations and deaths. That's what disease models are designed to do.
"We do acknowledge however that it's important to consider, not only direct losses from COVID, but also economic losses as a result of lockdown," says epidemiologist and JUNIPER member Mike Tildesley of the University of Warwick. At the Newton Gateway event Tildesley presented a tentative approach to how this might be done. As a measure of the economic dimension Tildesley and his colleagues used gross domestic product (GDP) and, as horrible as it might sound, assumptions on how much society as a whole might be willing to pay for an amount of healthy life saved (to be precise, for avoiding the loss of a quality adjusted life year, also called a QALY).
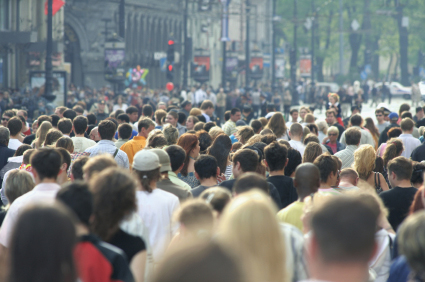
The dynamics of a disease depends on people's behaviour.
To show how these economic metrics could be taken into account, the team focused on circuit-breaker lockdowns as they were proposed in autumn 2020. They used the Warwick model, already mentioned above, to predict how much time such a circuit-breaker lockdown would have bought us in terms of temporarily reverting the exponential growth of cases.
But rather than just simulating the effect of one circuit-breaker of a given length and intensity, the team simulated a collection of circuit-breakers of varying timing, intensity and also frequency. They then showed how from this set of scenarios you can then pick one that minimises economic losses (measured by a combination of GDP loss and cost per QALY saved) based on a given willingness to pay for each QALY saved. The choice can also be constrained by requiring that the NHS must not be overwhelmed.
The idea, Tildesley points out, is only scratching the surface of the problem of how to incorporate economic concerns into modelling. GDP may not be the best measure to use here, and other economic considerations might come into play. But it's again a proof of concept. The approach suggests how policy makers might explore the economic impact of different lockdown policies and then pick one that's acceptable both in terms of public health and in terms of the economy. You can hear Tildesley talk about this work in our podcast.
Saving lives through partnerships
The idea that behavioural scientists need to be involved in COVID-19 response isn't new. Indeed SPI-M is complemented by SPI-B, the Independent Scientific Pandemic Insights Group on Behaviours.
SPI-M and SPI-B have been communicating throughout the pandemic, with revelatory consequences. "Working with the researchers on SPI-B has been an absolute eye-opener and I really hope those collaborations are going to persist long past this pandemic," Julia Gog of the University of Cambridge, who is a member of SPI-M and co-head of JUNIPER, told us in a podcast interview.
Brooke Rogers, mentioned at the beginning of this article, is a member of SPI-B and agrees. "Working with the modellers has been such an interesting process. I think we have the potential to save lives by building partnerships further upstream and insuring those partnerships are there during an extreme event. We are only going to make one another more effective," she said in her talk at the Newton Gateway event.
The event, which comprised many more speakers and topics than mentioned here, has helped crystallise what needs to be done next. One important task is to figure out what kind of data modellers would need from the social and behavioural sciences and establish the relevant data streams. Another is to think about how mathematical models are perceived by the public — do people feel they are useful and reliable — and to see how the public could be involved in modelling (see here for work that has already been done on this idea).
Crucially, says co-organiser Ed Hill, we need to keep the dialogue going. "It's important to nurture these initial conversations to enable us to highlight areas where collaboration might happen."
About this article
The Modelling behaviour to inform policy for pandemics event took place at the Newton Gateway for Mathematics in November 2021.
Marianne Freiberger is Editor of Plus. She would like to thank Ed Hill, a post-doctoral researcher at the University of Warwick and a member of the Zeeman Institute for Systems Biology & Infectious Disease Epidemiology Research (SBIDER) and the JUNIPER consortium, for his help in writing this article.
This article was produced as part of our collaborations with JUNIPER, the Joint UNIversity Pandemic and Epidemic Response modelling consortium, and the Isaac Newton Institute for Mathematical Sciences (INI).
JUNIPER comprises academics from the universities of Cambridge, Warwick, Bristol, Exeter, Oxford, Manchester, and Lancaster, who are using a range of mathematical and statistical techniques to address pressing question about the control of COVID-19. You can see more content produced with JUNIPER here.
The INI is an international research centre and our neighbour here on the University of Cambridge's maths campus. It attracts leading mathematical scientists from all over the world, and is open to all. Visit www.newton.ac.uk to find out more.
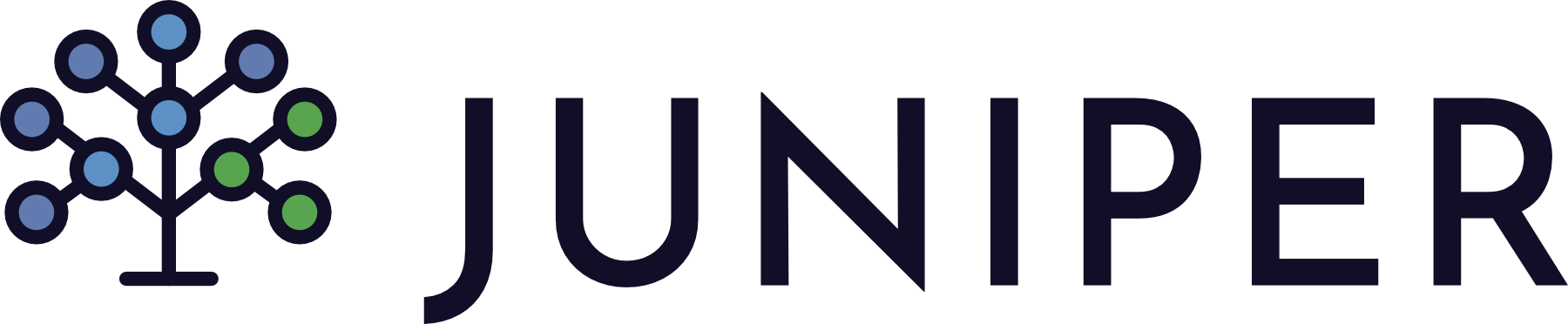
