
When you're in a situation of conflict and stress it's easy to become defensive of your views and blind to criticism, or else to defer to those with the loudest voice. These aren't the best ways of operating, but they're natural responses. To avoid them and reach a consensus, you need some sort of a strategy.
An interesting approach here is a strategy that's been dubbed Tell me why I'm wrong. It emerged as a modus operandi of SPI-M-O, a subgroup of the Scientific Advisory Group for Emergencies (SAGE) responsible for epidemiological modelling. The group's work was vital in formulating expert scientific advice for the UK government during the COVID-19 pandemic — advice which, whether it was taken or not, proved remarkably robust (see here for an assessment by one of the research groups involved in SPI-M-O).
Many SPI-M-O members have already been honoured individually for their work, but the team as a whole were recently awarded the prestigious Weldon Memorial Prize by the University of Oxford. It's the first time in its 111 year history that the prize was given, not to an individual, but to a group of people for a combined effort.
There's none to rule them all
SPI-M-O was the operational incarnation of the permanent group SPI-M, brought into being for the COVID-19 pandemic and disbanded in March 2022. The acronym SPI-M stands for Scientific Pandemic Influenza Group on Modelling.
The group was chaired by Graham Medley and Angela McLean, and comprised over 100 members. Most were academics, but representatives of government agencies, such as UKHSA, were also included. The advice that SPI-M-O fed to SAGE to pass on to the government was based on mathematical models that can simulate the spread of a disease (find out more about such models here).
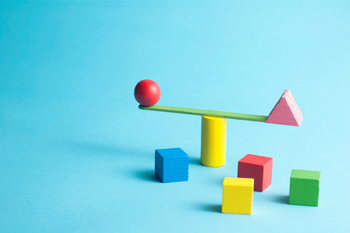
Consensus can be hard to find when there are many different models involved.
While most of us became aware as the pandemic unfolded that maths is a vital tool in understanding this spread, what is less well-known is that there isn't a single mathematical model to rule them all. At any one point in time, SPI-M-O were looking at a range of models developed by their members. Some were large-scale models designed to assess what the future had in store for the nation as a whole. Others were developed to look at particular aspects of the pandemic: to assess the effect of household bubbling, for example, or to predict what will happen to the R number in different scenarios.
Different models never agree 100%. A mathematical model is always a simplification of reality, especially when messy things like human behaviour and biology are involved. Models are based on sets of assumptions that usually turn out to be inaccurate, or too simplistic, and can impact the model's output in subtle ways.
All this doesn't mean that the mathematical models are useless — even the simplest of models can give valuable insights without needing to be accurate on every detail. It does mean, however, that you need to be aware of the limitations of a model, scrutinise its assumptions, and make sure you're clear about the uncertainties involved when you talk about your model's results.
While there are rigorous techniques for dealing with certain types of modelling uncertainties (see here for an example), the best way of understanding what a model is telling you is to put it into a broader context of other models. But the task that faced SPI-M-O went further than that: apart from comparing models they also had to formulate robust scientific advice based on a multitude of models with potentially conflicting results. This is where Tell me why I'm wrong became incredibly useful.
Kicking the wheels
"Tell me why I'm wrong - or TMWIW - was a code of language used very often in SPI-M-O," says senior member Julia Gog who also co-leads the JUNIPER modelling consortium. "No one quite knows when it started but it was certainly being used by February 2020, and both Graham Medley and Angela McLean were clearly leading on its usage." TMWIW means that once someone has presented their work or point of view, they actively encourage everyone else to pick it apart. "A similar though gentler version is phrase 'kick the wheels': if you buy a new car, you go and kick the wheels. You are not trying to break it, you're trying to see if there's a problem."
There are many examples of when TMWIW helped the group discover things that otherwise might have been lost, says Gog. One is that quite often research groups within SPI-M-O that were focussing on COVID cases got a very different picture from their work than groups that were focussing on hospitalisations and deaths. Rather than arguing about who's right and who's wrong, it's more instructive to try and figure out where the difference might come from. It may, for example, be an indication that something has changed about the severity of the disease, so the relationship between the number of people who caught it and the number of people who went to hospital or died changed.
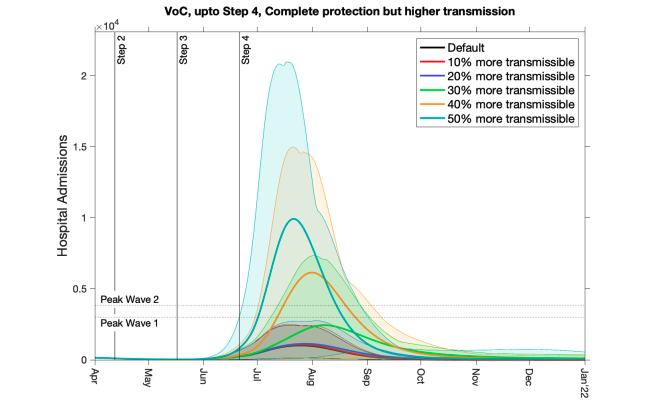
The typical output of a model. It was produced by a team from the University of Warwick in May 2021 to understand the threat of the delta variant, showing projected hospital admissions for a novel variant with higher transmission rates. Figure from Road Map Scenarios and Sensitivity: Steps 3 and 4 by Ed Hill and colleagues, published 5 May 2021.
Flattening the hierarchy
There are various useful things that TMWIW does, says Gog. One is to flatten the hierarchy. "The nature of the emergency brought together a range of researchers to SPI-M-O including both very senior and very junior scientists. Academe is fond of its hierarchical structures and so is government, and here we have the two sitting on the same committee. Especially under the high-pressure circumstances, there’s the risk that junior staff don’t feel able to speak out in disagreement. But as soon as someone they see as senior says 'tell me why I'm wrong', that flattens the human dynamics - it says that disagreement is welcomed. Working in this way requires trust in all directions, but leads to a more collaborative outcome"
Note that there's a "why" in Tell me why I'm wrong, rather than an "if". The difference is significant. Because the little word of "why" suggests, not that something could be wrong but that something's definitely wrong, it opens up the field even for junior people to speak up.
Being human
In science, the TWMIW attitude isn't new. People do science because they are curious about the world, so an ideal scientist should always welcome a better description of it than their own, or something that contradicts their working hypothesis. "Indeed, science progresses by proving itself wrong," says Medley. "Newton's theories stood for a long time until Einstein pointed out that they were wrong (at a galactic scale). Biochemistry textbooks have a new edition every year because what was in the last edition is now wrong."
The situation that faced SPI-M-O members, though, was different from the normal pursuit of science they were used to. Not only because doing science is very different from using science to form evidence for decision making, but also because they were people living through an unprecedented crisis.
"The ideal scientist is this virtuous being that changes their mind according to the evidence without any preconceived biases," says Gog. "But during the COVID-19 emergency, we are just human beings under all kinds of unusual pressures, and of course we and our loved ones were experiencing the pandemic ourselves, and many scientists were stating their opinions very publically, so neutral objectivity was at risk. TMWIW brings it back by signalling that I'm going to be curious, I'm going to be ok about my worldview pivoting today, and I’m ready to learn something new." This, says Gog, is another welcome effect of the strategy.
Robustness through diversity
A third welcome effect is to lend a platform to diversity — the diversity of the people in the group, in how they view the group and go about their work, and also the diversity of the mathematical models. "Groups under pressure can polarise very quickly around one very loud and over-confident voice. The illusion of certainty can be very appealing, and that risks the whole group being biassed the same way." TMWIW is a way of breaking out of this danger of groupthink, and brings back in the range of different voices in the group, making the most out of its diversity.
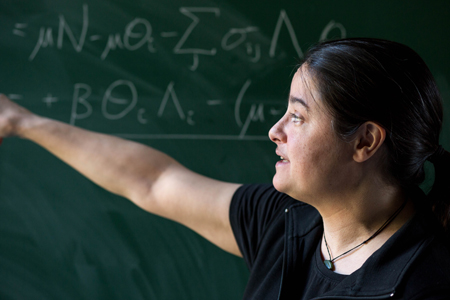
Julia Gog. Photograph by Henry Kenyon.
Fourthly, and crucially, TMWIW lent robustness to advice SPI-M-O issued to the government. "We wanted to solve the problems we faced as quickly as possible, but we also wanted to hear about the things that didn't fit, even if it led to a complicated working picture." says Gog. "Then our consensus statements included some of those uncertainties — we'd say, 'here's what we think as a general consensus, however, this would change if a particular assumption changes', or 'one group found a result that doesn't fit, using a different approach'."
This meant that, contrary to some popular belief, the advice issued wasn't presented as ironclad certainty, but included a range of scenarios and highlighted the most important assumptions they were based on. "The consensus was a consensus on the uncertainty as much as of a central view," says Medley. "Getting a consensus on what we don’t know, as much as what we do know, is difficult, and TMWIW was a critical part of that process."
Even the very act of witnessing discussions, says Medley, allowed members of government to better get to grips with the science. "It is very useful for government people to see the scientists telling each other why they are wrong," says Medley. "They learnt a lot from the discussion about the models - far more than if we had been trying to find the answer rather than presenting several answers and their associated weaknesses."Beyond COVID?
The TMWIW strategy requires some level of trust between the people in the group which, Medley says, may not be present in the same way in peacetime. "The culture on SPI-M-O evolved so that people would say 'tell me why I am wrong' and mean it. It’s easier to do this in the SPI-M-O context because there is a higher, common goal, but may be harder to maintain outside where academic success depends on people convincing others that they are right."
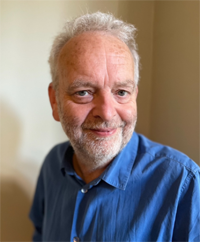
Graham Medley.
Nevertheless, Gog and Medley think the strategy is well worth keeping. "You already get it a little bit in normal research patterns but on much slower and subtler time scales," Gog says. "We're going to keep this way of working, it's really powerful for robust results, and just leads to a better way of making the most of having multiple research teams. In general, preserving the TMWIW approach with a large group in an emergency is something I'd advocate anyway."
The UK government's handling of the COVID-19 endemic has received much criticism, and is currently being scrutinised by the COVID-19 inquiry. But when it comes to the scientific, rather than policy, response the country certainly set an example. "No matter what people think of the decisions made during the pandemic, the amount of work and expertise that people put in to enable the modelling evidence was awe-inspiring," Medley tweeted in October 2022.
In part, says Gog, this is due to the collective effort that allowed a multiplicity of approaches. The fact that the prestigious Weldon Prize was awarded to the entire team reflects this.
About this article
Julia Gog is Professor of Mathematical Biology at the University of Cambridge and co-leads the JUNIPER modelling consortium.
Graham Medley is Professor of Infectious Disease Modelling at the London School of Hygiene and Tropical Medicine and co-led SPI-M-O.
Marianne Freiberger is Editor of Plus. She interviewed Gog in December 2022 and corresponded with Medley in January 2023.
This article is part of our collaboration with JUNIPER, the Joint UNIversity Pandemic and Epidemic Response modelling consortium. JUNIPER comprises academics from the universities of Cambridge, Warwick, Bristol, Exeter, Oxford, Manchester, and Lancaster, who are using a range of mathematical and statistical techniques to address pressing questions about the control of COVID-19. You can see more content produced with JUNIPER here.
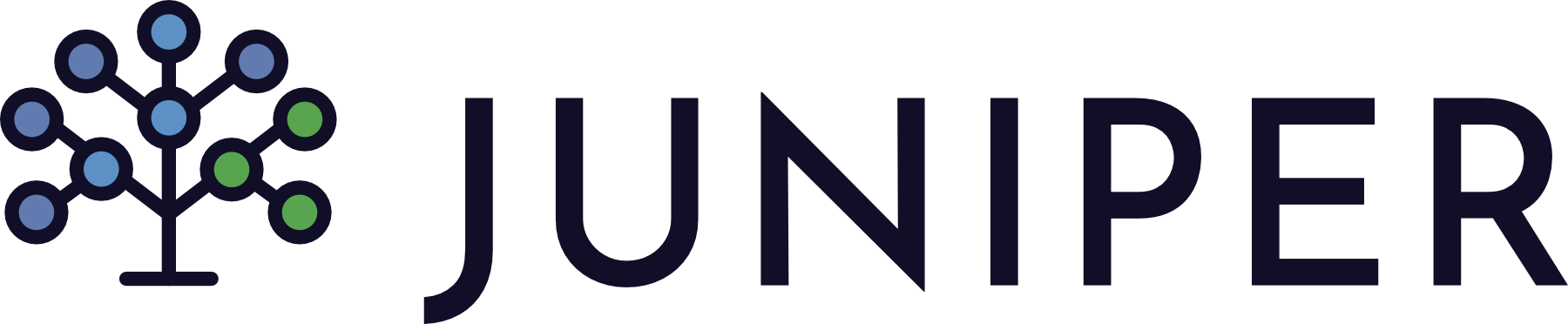
Comments
Great idea
Love this - I think a similar approach can be used to make the most of diversity of thought in other contexts too. I'll be seeing how I can apply lessons from this article in my field of Software Engineering - perhaps in design reviews or code reviews.
Logic applied to science
The simple approach is to claim that theories must always depend on logical analytic thinking. However, I seems to me that in my subject of macroeconomics this is not necessarly true. The macroeconomics twin axioms of Henry George are most pertinant there. "Mankind seeks to satisfy his/her desires with the least exertion." Yet "Mankind's desires are unlimited". These are opposite trends, but which must apply simultaneously. So it becomes logical to be illogical too!