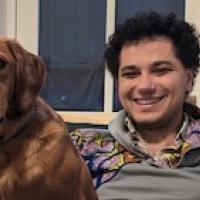
Kweku Abraham is an early career researcher here at the Department of Pure Mathematics and Mathematical Statistics (DPMMS) at the University of Cambridge. He is also a member of Maths4DL, a research project which investigates deep learning we're proud to be collaborating with.
As part of our series of interviews with early career researchers, Kweku tells us why he enjoys statistics, what life as an early career researcher is like, and about a favourite mathematical moment.
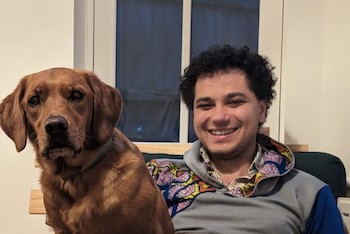
Kweku Abraham
I work in statistical theory. That means I check that the ways people try to make sense of data will work, in that they'll converge on the truth as more and more data is fed in.
Mostly I've done this for Bayesian methods. These allow you to take the knowledge you (think you) have about something (your prior belief) and update it based on observations, using a theorem called Bayes' rule. So I focus on the posterior beliefs – the knowledge you have after updating – to check they close in on the truth. This is well understood in what are called parametric models, when the data follows a known probability distribution – for example, you know data follows a normal distribution and it's only some parameters, like the mean and variance, that are unknown. My study has been in non-parametric, or infinite-dimensional, statistics, where there's a whole unknown function governing the data.
In my undergraduate studies I always particularly enjoyed analysis and the study of limits – the analytic way of thinking, with epsilons and deltas, just made sense to me. I also enjoyed probability and statistics courses. So when I learned about asymptotic statistics and the chance to prove limit theorems for statistical estimators, it seemed a natural area to work in. It helps that it's a way of using my analysis skills to solve at least somewhat tangible problems!
Work habits have changed somewhat since the pandemic, but when I meet my research group it’s always nice to be reminded of what personable and bright people I'm surrounded by! I'm hoping to get lunch with colleagues more often over the next year, have some time for informal maths chat as well as just socially catching up. I'm also excited by a new lunchtime seminar that's started up in the Statslab at DPMMS for PhDs and postdocs!
I've been doing a lot of undergraduate supervisions at the moment, so a typical day might involve some marking time, and a couple of hours spent with two undergraduate students talking through problems from one of their courses. My professor wrote the third year statistics theory course that I regularly supervise so the material is right up my street!
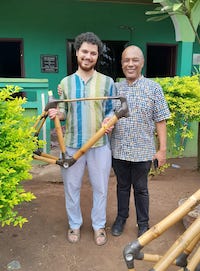
Kweku, his dad, and a bamboo bike frame at a business in rural Ghana his dad advises.
Other than that there's a lot of time reading papers to try to make sense of what they do and whether it can be adapted to solve whatever problem I'm working on, a lot of editing work (getting the main content of a paper done is really only half the battle: writing it all up nicely for submitting to a journal takes a long time), and some pen-and-paper puzzling over what conditions I might need for whatever I'm trying to prove. I’m hoping to get into slightly more directly practical things so in the near future days may involve running analysis on real and simulated data sets.
One of my favourite mathematical moments was in the same third year statistics course I often teach now. Up to this point, it was not clear to me that statistics was "doing anything", other than providing heuristics. But a key result [in the course] is the Cramér-Rao theorem, which provides a lower bound on the quality of statistical estimators.
For example, suppose you want to know how many posts arrive on your social media feed per minute on average. The arrival of posts is governed by a Poisson distribution, so your task is to estimate the mean of the distribution. It makes sense to do this by observing your feed for a while and using the average arrival per minute (the sample mean) as an estimator for the distribution mean. But it's a fact about the Poisson distribution that the spread of the data (the variance) is equal to the mean, so we could also use the sample variance as an estimator. Could we improve on the sample mean by using information from the spread of the data? The Cramér-Rao theorem allows us to answer this question, showing that no unbiased estimator can outperform the sample mean
That means there's hope of doing something definitive in statistics: rather than having to perpetually come up with algorithms and always harbour the possibility we could be doing better, we can try to find best-possible algorithms. I didn't know it at the time, but my love of this theorem helped set me on the path to my research today.
About this article
This article is part of our collaboration with the Mathematics for Deep Learning (Maths4DL) research programme, which brings together researchers from the universities of Bath and Cambridge, and University College London. Maths4DL aims to combine theory, modelling, data and computation to unlock the next generation of deep learning. You can see more content produced with Maths4DL here.
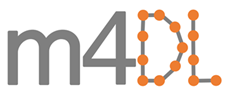